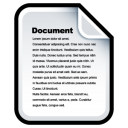
DC Field | Value | Language |
---|---|---|
dc.contributor.advisor | Tiedemann, Tim | - |
dc.contributor.author | Tuleweit, Marcel | - |
dc.date.accessioned | 2022-02-21T11:18:40Z | - |
dc.date.available | 2022-02-21T11:18:40Z | - |
dc.date.created | 2020-03-03 | - |
dc.date.issued | 2022-02-21 | - |
dc.identifier.uri | http://hdl.handle.net/20.500.12738/12466 | - |
dc.description.abstract | Die Eignung von Machine Learning Verfahren im Bereich der Medizin ist bereits bewiesen. Inwiefern sich ein einzelnesVerfahren für die Problemlösung des jeweiligen Anwendungsbereiches eignet, ist meist von dem zu lösenden Problem abhängig und nicht zuletzt auch von dem Charakteristikum des gewählten Verfahrens. Im Bereich der Elektroenzephalographie ist diese Eignung bereits durch viele Verfahren gezeigt worden. Die involvierten Machine Learning Verfahren gilt es in einer hinreichenden Analyse und anhand eines Beispiels gegenüberzustellen und ihre Vor- und Nachteile näher zu beleuchten. | de |
dc.description.abstract | The suitability of machine learning procedures in the field of medicine has already been proven. The extent to which a single method is suitable for solving the problem of the respective application area usually depends on the problem to be solved and, last but not least, on the characteristic of the chosen method. In the field of electroencephalography, this suitability has already been demonstrated by many procedures. The machine learning procedures involved must be compared in a sufficient analysis and on the basis of an example and their advantages and disadvantages must be examined in more detail. | en_US |
dc.language.iso | de | en_US |
dc.rights.uri | http://rightsstatements.org/vocab/InC/1.0/ | - |
dc.subject | Maschinelles Lernen | en_US |
dc.subject | Elektronenzephalographie | en_US |
dc.subject | Deep Learning | en_US |
dc.subject | Clusteranalyse | en_US |
dc.subject | Hyperparameter Tuning | en_US |
dc.subject.ddc | 004: Informatik | en_US |
dc.title | Klassifikation auf Basis von EEG-Daten, automatisiert durch maschinelles Lernen | de |
dc.type | Thesis | en_US |
openaire.rights | info:eu-repo/semantics/openAccess | en_US |
thesis.grantor.department | Fakultät Technik und Informatik | en_US |
thesis.grantor.department | Department Informatik | en_US |
thesis.grantor.universityOrInstitution | Hochschule für Angewandte Wissenschaften Hamburg | en_US |
tuhh.contributor.referee | Clemen, Thomas | - |
tuhh.identifier.urn | urn:nbn:de:gbv:18302-reposit-140551 | - |
tuhh.oai.show | true | en_US |
tuhh.publication.institute | Fakultät Technik und Informatik | en_US |
tuhh.publication.institute | Department Informatik | en_US |
tuhh.type.opus | Bachelor Thesis | - |
dc.type.casrai | Supervised Student Publication | - |
dc.type.dini | bachelorThesis | - |
dc.type.driver | bachelorThesis | - |
dc.type.status | info:eu-repo/semantics/publishedVersion | en_US |
dc.type.thesis | bachelorThesis | en_US |
dcterms.DCMIType | Text | - |
tuhh.dnb.status | domain | - |
item.grantfulltext | open | - |
item.creatorGND | Tuleweit, Marcel | - |
item.cerifentitytype | Publications | - |
item.creatorOrcid | Tuleweit, Marcel | - |
item.advisorGND | Tiedemann, Tim | - |
item.languageiso639-1 | de | - |
item.openairecristype | http://purl.org/coar/resource_type/c_46ec | - |
item.fulltext | With Fulltext | - |
item.openairetype | Thesis | - |
Appears in Collections: | Theses |
Files in This Item:
File | Description | Size | Format | |
---|---|---|---|---|
Bachelorthesis-AI-19-20_Tuleweit.pdf | 1.17 MB | Adobe PDF | View/Open |
Note about this record
Export
Items in REPOSIT are protected by copyright, with all rights reserved, unless otherwise indicated.