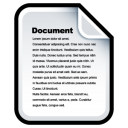
DC Field | Value | Language |
---|---|---|
dc.contributor.advisor | Margaritoff, Petra | - |
dc.contributor.author | Montenegro Martinez, Larissa Muriel | - |
dc.date.accessioned | 2022-12-02T14:48:04Z | - |
dc.date.available | 2022-12-02T14:48:04Z | - |
dc.date.created | 2021 | - |
dc.date.issued | 2022-12-02 | - |
dc.identifier.uri | http://hdl.handle.net/20.500.12738/13400 | - |
dc.description.abstract | As the access to more processing resources has increased over the recent decades, the number of studies and development of Deep Learning (DL) algorithms for cardiac arrhythmia classification tasks have grown. The success of arrhythmia classification tasks with Machine Learning (ML) algorithms is based on the extraction of features from Electrocardiography (ECG) signals. The feature engineering process requires prior knowledge of the ECG processing techniques, as well as a level of understanding of ECG signal interpretation. Deep Neural Networks (DNN) bypass the process of hand-crafting features extraction, as their hidden layers perform the task of learning and extracting specific features from raw ECG signals. The deeper a network is, the more specific and complex are the extracted features. The main goal of this thesis is to explore ML techniques for automatic classification of arrhythmia from ECG signals. Deep features extracted from time-series ECG signals have not been extensively studied in arrhythmia classification tasks. Towards this end, two approaches for feature extraction will be addressed: (1) human-assisted feature extraction approaches, based on traditional signal processing techniques (feature engineering); and (2) data representation learning based on Deep Learning (DL) methods by exploring 1-dimensional Convolutional Neural Networks (CNN) as feature provider. The results show classification metrics achieved in the task of rhythm classification by means of a Convolutional Neural Networks (CNN) and a Support Vector Machine (SVM) as ML classifier. Both methods were trained with the same dataset of cardiac rhythms consisting of two type two balanced rhythms categories: normal sinus and other rhythms. The 34 layer 1-dimensional CNN achieved the best validation metrics for classification, with an overall precision 93.94%, 93.07% accuracy and F1-score 93.05 %. The second best classification metrics were achieved by two combined hand-crafted featured extracted methods: Wavelet transform and R-Peak Interval features using the SVM classifier, which achieved an overall precision of 89.00 %, accuracy of 98.00 % and F1-score of 92%. Although, the classification accuracy values between the two methods seems really close, for classification task we should always take into account the precision value, as it is the one that tell us the percentage of rhythms being correctly predicted. In addition, features from the first convolutional layer of the CNN were extracted to be input to the SVM. However, CNN features cannot be used by SVNs as is and required human-assisted feature engineering to convert the them into a SVM-compatible format using a combined wavelet and power spectral density feature processing. Repeatedly low accuracy values peaking at 67.33%, along with the high computational costs of the CNN and human intervention rendered the use of a CNN for this task impractical and redundant. | en |
dc.language.iso | en | en_US |
dc.subject.ddc | 600: Technik | en_US |
dc.title | Human-assisted vs deep learning feature extraction : An evaluation of ECG features extraction methods for arrhythmia classification using machine learning | en |
dc.type | Thesis | en_US |
openaire.rights | info:eu-repo/semantics/openAccess | en_US |
thesis.grantor.department | Fakultät Life Sciences | en_US |
thesis.grantor.department | Department Medizintechnik | en_US |
thesis.grantor.universityOrInstitution | Hochschule für Angewandte Wissenschaften Hamburg | en_US |
tuhh.contributor.referee | Fred, Ana | - |
tuhh.identifier.urn | urn:nbn:de:gbv:18302-reposit-151796 | - |
tuhh.oai.show | true | en_US |
tuhh.publication.institute | Fakultät Life Sciences | en_US |
tuhh.publication.institute | Department Medizintechnik | en_US |
tuhh.type.opus | Masterarbeit | - |
dc.type.casrai | Supervised Student Publication | - |
dc.type.dini | masterThesis | - |
dc.type.driver | masterThesis | - |
dc.type.status | info:eu-repo/semantics/publishedVersion | en_US |
dc.type.thesis | masterThesis | en_US |
dcterms.DCMIType | Text | - |
tuhh.dnb.status | domain | - |
item.openairecristype | http://purl.org/coar/resource_type/c_46ec | - |
item.fulltext | With Fulltext | - |
item.grantfulltext | open | - |
item.openairetype | Thesis | - |
item.creatorGND | Montenegro Martinez, Larissa Muriel | - |
item.languageiso639-1 | en | - |
item.creatorOrcid | Montenegro Martinez, Larissa Muriel | - |
item.cerifentitytype | Publications | - |
item.advisorGND | Margaritoff, Petra | - |
Appears in Collections: | Theses |
Files in This Item:
File | Description | Size | Format | |
---|---|---|---|---|
MontenegroMartinezLarissaMurielMA_geschwärzt.pdf | 25.3 MB | Adobe PDF | View/Open |
Note about this record
Export
Items in REPOSIT are protected by copyright, with all rights reserved, unless otherwise indicated.