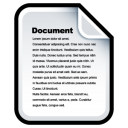
Title: | Untersuchung von Active Learning Methoden für BERT-Modelle | Language: | German | Authors: | Wischhusen, Jonathan | Keywords: | Active Learning; BERT; Textklassifikation | Issue Date: | 12-Jan-2024 | Abstract: | Automatisierte Textklassifikation ist für viele praktische Anwendungen ein aussichtsreiches Analyse- und Moderationsinstrument. In der Praxis steht Textklassifikation jedoch oft teuren Annotationskosten und einem Ungleichgewicht der Klassen in den Trainingsdaten gegenüber. Active Learning beschreibt ein Paradigma, dass die Kosten für die Annotation signifikant senken kann, indem über mehrere Iterationen geeignete Daten gezielt annotiert werden. In Verbindung mit vortrainierten Sprachmodellen, wie BERT und seinen Variationen, ist Active Learning bisher wenig untersucht. Diese Arbeit untersucht verschiedene Active Learning Methoden für BERT-Modelle unter dem Problem der Mehr-Klassen-Textklassifikation. Der Fokus liegt auf Szenarien mit praxisnahen Startmengen und ihre Auswirkung für vielfältige Datensätze. Die Ergebnisse zeigen, dass Discriminate Active Learning im Umfeld der Untersuchung als einzige Methode über die Modelle und Daten hinweg signifikant besser ist als der Zufall. Andere Methoden sind in der Regel nicht besser als der Zufall, außer in einigen praxisnahen Situationen. Die Arbeit gewährt ebenfalls einen Einblick in den Einsatz des Menschen als Orakel. Durch eine Benutzerstudie wird beobachtet, dass für ein kleines Annotationsbudget Menschen eine konstante Leistung zeigen und Domänenwissen auch bei einfachen Kategorien hilfreich ist. Automated text classification is a promising analysis and moderation tool for many practical applications. In practice, text classification often faces expensive annotation costs and class imbalance in the training data. Active Learning describes a paradigm that can significantly reduce annotation costs by selectively annotating the most suitable data over multiple iterations. In conjunction with pre-trained language models, such as BERT and its variations, Active Learning has been little studied. This work investigates Active Learning for BERT models under the problem of multi-class text classification. The focus is on scenarios with practical warmstart sets and their impact for diverse datasets. The results show that Discriminate Active Learning is the only method that significantly outperforms the random baseline across models and datasets in the setting of the study. Other methods are outperfoming the baseline only for some real-world scenarios. The work also provides insight into the use of humans as an oracle. A user study concludes that humans show consistent performance over a small annotation budget and that domain knowledge is helpful even for simple categories. |
URI: | http://hdl.handle.net/20.500.12738/14558 | Institute: | Fakultät Technik und Informatik Department Informatik |
Type: | Thesis | Thesis type: | Master Thesis | Advisor: | Zukunft, Olaf | Referee: | Sarstedt, Stefan |
Appears in Collections: | Theses |
Files in This Item:
File | Description | Size | Format | |
---|---|---|---|---|
Masterthesis.pdf | 1.65 MB | Adobe PDF | View/Open |
Note about this record
Export
Items in REPOSIT are protected by copyright, with all rights reserved, unless otherwise indicated.