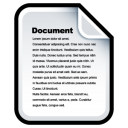
DC Field | Value | Language |
---|---|---|
dc.contributor.advisor | Zukunft, Olaf | - |
dc.contributor.author | Tarabih, Dina | - |
dc.date.accessioned | 2024-03-01T14:03:35Z | - |
dc.date.available | 2024-03-01T14:03:35Z | - |
dc.date.created | 2021-05-20 | - |
dc.date.issued | 2024-03-01 | - |
dc.identifier.uri | http://hdl.handle.net/20.500.12738/14975 | - |
dc.description.abstract | Das Internet hat zu einer Zunahme elektronischer Online-Dokumente geführt. Dies hat Internetnutzern aufgrund der Informationsexplosion erschwert, relevante und genaue Informationen zu finden. Heutzutage wurden Techniken und Methoden des Text-Minings, maschinellen Lernens und der Computerlinguistik eingesetzt, um Big-Data zu verarbeiten. Diese Arbeit beschäftigt sich insbesondere mit dem Problem der Textähnlichkeit, eine wachsende Anwendung der Verarbeitung natürlicher Sprache, zur Optimierung der Suche von Dokumenten. Dabei werden vier Kombinationen verschiedener Feature-Extraktionstechniken TF-IDF, Word2Vec, Doc2Vec und BERT untersucht. Zusätzlich werden zwei Ähnlichkeitsalgorithmen Kosinusähnlichkeit und Word Mover’s Distance zur Berechnung der Ähnlichkeitswerte eingesetzt. Diese Methoden werden anhand eines Datensatzes getestet, der 16.876 Artikel aus der New York Times-Tageszeitung enthält. Infolgedessen werden Hypothesen auf Basis von initialen Ergebnissen erstellt. Diese werden durch weitere Experimente zur Bewertung der Plausibilität und Zuverlässigkeit der erzielten Ergebnisse getestet. | de |
dc.description.abstract | The Internet has led to an increase in electronic documents online. This has made it difficult for internet users to find relevant and accurate information due to the information explosion. Today, text mining, machine learning, and natural language processing techniques and methods have been used to process big data. This thesis deals in particular with the problem of text similarity, a growing application of natural language processing, for the optimisation of document search. Four combinations of different feature extraction techniques, TF-IDF, Word2Vec, Doc2Vec, and BERT, are examined. In addition, two similarity algorithms, Cosine Similarity and Word Mover’s Distance, are used to calculate the similarity values. These methods are tested on a data set containing 16,876 articles from the New York Times daily newspaper. As a result, hypotheses are created based on initial results. These are tested by further experiments to assess the plausibility and reliability of the results obtained. | en |
dc.language.iso | de | en_US |
dc.subject | Text-Mining | en_US |
dc.subject | Maschinelles Lernen | en_US |
dc.subject | Computerlinguistik | en_US |
dc.subject | Big-Data | en_US |
dc.subject | Textähnlichkeit | en_US |
dc.subject | Word2Vec | en_US |
dc.subject | Doc2Vec | en_US |
dc.subject | BERT | en_US |
dc.subject | Kosinusähnlichkeit | en_US |
dc.subject | Word Mover’s Distance | en_US |
dc.subject | New York Times | en_US |
dc.subject | Machine Learning | en_US |
dc.subject | Natural Language Processing | en_US |
dc.subject | Text Similarity | en_US |
dc.subject | Cosine Similarity | en_US |
dc.subject.ddc | 004: Informatik | en_US |
dc.title | Textähnlichkeit unter Verwendung von Ansätzen des maschinellen Lernens | de |
dc.type | Thesis | en_US |
openaire.rights | info:eu-repo/semantics/openAccess | en_US |
thesis.grantor.department | Fakultät Technik und Informatik | en_US |
thesis.grantor.department | Department Informatik | en_US |
thesis.grantor.universityOrInstitution | Hochschule für Angewandte Wissenschaften Hamburg | en_US |
tuhh.contributor.referee | Sarstedt, Stefan | - |
tuhh.identifier.urn | urn:nbn:de:gbv:18302-reposit-181163 | - |
tuhh.oai.show | true | en_US |
tuhh.publication.institute | Fakultät Technik und Informatik | en_US |
tuhh.publication.institute | Department Informatik | en_US |
tuhh.type.opus | Bachelor Thesis | - |
dc.type.casrai | Supervised Student Publication | - |
dc.type.dini | bachelorThesis | - |
dc.type.driver | bachelorThesis | - |
dc.type.status | info:eu-repo/semantics/publishedVersion | en_US |
dc.type.thesis | bachelorThesis | en_US |
dcterms.DCMIType | Text | - |
tuhh.dnb.status | domain | en_US |
item.openairecristype | http://purl.org/coar/resource_type/c_46ec | - |
item.fulltext | With Fulltext | - |
item.grantfulltext | open | - |
item.openairetype | Thesis | - |
item.creatorGND | Tarabih, Dina | - |
item.languageiso639-1 | de | - |
item.creatorOrcid | Tarabih, Dina | - |
item.cerifentitytype | Publications | - |
item.advisorGND | Zukunft, Olaf | - |
Appears in Collections: | Theses |
Files in This Item:
File | Description | Size | Format | |
---|---|---|---|---|
BA_Maschinelles Lernen.pdf | 3.3 MB | Adobe PDF | View/Open |
Note about this record
Export
Items in REPOSIT are protected by copyright, with all rights reserved, unless otherwise indicated.