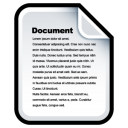
Title: | Explainability vs. Interpretability and methods for models’ improvement | Language: | English | Authors: | Tran, Thu Thao | Keywords: | Artificial Intelligence; XAI; Explainability; Interpretability | Issue Date: | 22-Mar-2024 | Abstract: | Artificial Intelligence is getting more and more involved in our daily lives, it is being used in almost every area nowadays, from medicinal treatment to autonomous driving. Therefore, another important aspect of artificial intelligence has surfaced in order to assist human in understanding why or how a machine concludes a decision. This aspect is called eXplainable Artificial Intelligence (XAI) or Interpretable Artificial Intelligence. However, it is important to distinguish the difference between “explainable” and “interpretable”. Until now, these two terms have been used interchangeably in most of the research papers, this paper attempts to analyze the differences between explainable and interpretable artificial intelligence and hopefully provide people a clear picture of how to use these terms correctly in the future. On the other hand, this paper also attempts to analyze different existing methods or techniques which improve the system’s explainability or interpretability. Depending on how deep the users would like to know about the systems they are working with, this paper will provide a more thorough overview when these methods are implemented. Künstliche Intelligenz wird immer mehr in unser tägliches Leben einbezogen und heutzutage in fast allen Bereichen eingesetzt, von medizinischer Behandlung zum autonomen Fahren. Aus diesem Grund haben Experten einen weiteren Aspekt der künstlichen Intelligenz entwickelt, um es verständlicher zu machen, warum oder wie eine Maschine eine Entscheidung trifft. Dieser Aspekt wird als eXplainable Artificial Intelligence (XAI) oder Interpretable Artificial Intelligence bezeichnet. Es ist jedoch wichtig, den Unterschied zwischen „erklärbar“ und „interpretierbar“ auseinander zu halten. Die beiden Begriffe wurden bisher in den meisten Forschungsarbeiten als synonym behandelt. In dieser Arbeit wird versucht, die Unterschiede zwischen erklärbarer und interpretierbarer künstlicher Intelligenz zu analysieren und dadurch den Menschen ein klares Bild darzustellen, wie die beiden Begriffe richtig verwendet werden sollten. Andererseits wird hier auch versucht, verschiedene vorhandene Methoden oder Techniken zu untersuchen, die die Erklärbarkeit oder Interpretierbarkeit des Systems verbessern. Es ist davon abhängig, wie viel die Benutzer über das System, mit denen sie arbeiten, erfahren oder verstehen möchten. Dafür bietet diese Arbeit einen genaueren Überblick über die Implementierung dieser Methoden. |
URI: | http://hdl.handle.net/20.500.12738/15293 | Institute: | Fakultät Technik und Informatik Department Informatik |
Type: | Thesis | Thesis type: | Bachelor Thesis | Advisor: | von Luck, Kai | Referee: | Draheim, Susanne ![]() |
Appears in Collections: | Theses |
Files in This Item:
File | Description | Size | Format | |
---|---|---|---|---|
BA_Explainability.pdf | 1.24 MB | Adobe PDF | View/Open |
Note about this record
Export
Items in REPOSIT are protected by copyright, with all rights reserved, unless otherwise indicated.