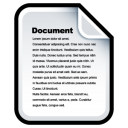
Title: | Machine-Learning-basierte Qualitätserkennung von Fitnessübungen auf Grundlage von Pose Estimation | Language: | German | Authors: | Bardtke, Leonard | Keywords: | Pose Estimation; Human Activity Recognition; Quantified Self; Zeitreihen; LSTM | Issue Date: | 17-May-2024 | Abstract: | Hilfe von Human Activity Recognition ist es möglich, die eigene Gesundheit im Auge zu behalten und Verletzungen zu vermeiden. Hierbei wird zwischen den beiden Haupttechniken der Datenerfassung im Gebiet der Human Activity Recognition differenziert. Zum einen handelt es sich dabei um den Bereich der Computer Vision und zum anderen um den Ansatz, welcher auf dem Verwenden von tragbaren Sensoren basiert. In dieser Arbeit erfolgt die Qualitätserkennung von Fitnessübungen anhand von Liegestützen, welche als Videodaten vorliegen. Um die zu analysierenden Parameter auf die Informationen zu reduzieren, die für die Qualitätserkennung relevant sind, erfolgte eine Reduzierung der Merkmale mit Hilfe von Pose Estimation. Diese liefert die Koordinaten bestimmter Körperschlüsselpunkte (Keypoints), mit denen das Erstellen eines Skeletts der trainierenden Person möglich ist. Bei den Keypoints handelt es sich um Gelenke und Körperteile, wie den Ellenbogen oder der Hüfte, wobei die Anzahl der zu erkennen Keypoints je nach Modell variiert. Um die Qualität der Liegestütze zu bestimmen, wurden Machine Learning in Form von Long Short-Term Memory (LSTM) verwendet. Neben LSTMs, welche die Koordinaten der Keypoints für die Bestimmung der Ausführungsqualität verwenden, wurden zudem Modelle trainiert, die die Klassifikation anhand der Winkel zwischen ausgewählten Körperteilen vornehmen. In dieser Arbeit konnte gezeigt werden, dass sich die Winkeldaten für eine Klassifikation deutlich besser eignen. Die binäre Klassifizierung, welche lediglich zwischen einer korrekten und einer nicht korrekten Ausführung unterscheidet, wies eine Testgenauigkeit von 91,5% auf. Um die Art der Fehlausführung spezifizieren zu können wurde zudem eine Mehrklassen-Klassifzierung untersucht. Hierbei gelang es die Qualität der Ausführung lediglich mit einer Genauigkeit von 60,71% zu bestimmen. Dies ist zum einen auf die Ungleichverteilung des Datensatzes zurückzuführen und zum andern auf die Schwierigkeit, zwischen den definierten Klassen zu unterscheiden. Physical activity is an important part of a healthy lifestyle. With the help of human activity recognition, it is possible to keep track of one’s health and avoid injuries. There are two main techniques of data acquisition in the field of human activity recognition. On the one hand there is the field of computer vision and on the other hand the approach, which is based on the use of wearable sensors. In this work, the quality of fitness exercises is identified using push-ups, which are available as video data. Pose estimation is used to reduce the parameters to the information relevant for quality recognition. This provides the coordinates of certain keypoints of the body with which it is possible to create a skeleton of the person being trained. The keypoints are joints and parts of the body, such as elbows or the hip, whereby the number of keypoints that can be recognized varies depending on the model. Machine learning in the form of long short-term memory (LSTM) was used to determine the quality of the push-ups. In addition to LSTM-model, which uses the coordinates of the keypoints to determine the quality of the execution, models were also trained to perform the classification based on the angles between selected body parts. This work has shown that the angle data are much better suited for classification. The binary classification, which only distinguishes between correct and incorrect execution, had a test accuracy of 91.5%. To be able to specify the type of incorrect execution, a multi-class classification was also examined. It was only possible to determine the quality of the execution with an accuracy of 60.71%. On the one hand, this is due to the unequal distribution of the data set and, on the other hand, to the difficulty of distinguishing between the defined classes. |
URI: | http://hdl.handle.net/20.500.12738/15755 | Institute: | Fakultät Technik und Informatik Department Informatik |
Type: | Thesis | Thesis type: | Bachelor Thesis | Advisor: | Pareigis, Stephan ![]() |
Referee: | von Luck, Kai |
Appears in Collections: | Theses |
Files in This Item:
File | Description | Size | Format | |
---|---|---|---|---|
BA_Machine-Learning-basierte-Qualitätserkennung.pdf | 4.12 MB | Adobe PDF | View/Open |
Note about this record
Export
Items in REPOSIT are protected by copyright, with all rights reserved, unless otherwise indicated.