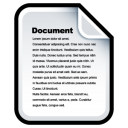
Title: | Untersuchung der Auswirkung von Skalierung vor dem SoftMax Layer auf die Robustheit eines Neuronalen Netzes | Language: | German | Authors: | Schradick, Lara Felina | Keywords: | Neuronale Netze; Robustheit; Maschinelles Lernen; SoftMax; Skalierungseffekt; Label Noise; Vanishing Gradients; Batch Normalisation; Neural Networks; Stability; Machine Learning; Softmax; scaling; label noise; vanishing Gradients; batch Normalisation | Issue Date: | 2-Aug-2024 | Abstract: | Vergleich von drei verschiedenen Ansätzen zur Stabilisierung neuronaler Netze gegen zwei unterschiedliche Arten von inkorrekt gelabelten Trainingsdaten. Dabei wollen alle drei Ansätze durch Abgrenzung von Logits ein besseres Trainingsergebnis erzielen. Zwei der Ansätze enthalten extra Schritte um Vanishing Gradients vorzubeugen. Comparison of three different approaches to stabilize neural networks against two different types of incorrectly labeled training data. All three approaches aim to achieve a better training result by better differentiating logits. Two of the approaches include extra steps to prevent vanishing gradients. |
URI: | https://hdl.handle.net/20.500.12738/16136 | Institute: | Fakultät Technik und Informatik Department Informatik |
Type: | Thesis | Thesis type: | Bachelor Thesis | Advisor: | Stelldinger, Peer ![]() |
Referee: | Buth, Bettina |
Appears in Collections: | Theses |
Files in This Item:
File | Description | Size | Format | |
---|---|---|---|---|
BA_Skalierung auf dem SoftMax Layer_Neuronales Netz.pdf | 1.48 MB | Adobe PDF | View/Open |
Note about this record
Export
Items in REPOSIT are protected by copyright, with all rights reserved, unless otherwise indicated.