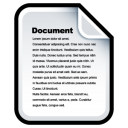
DC Element | Wert | Sprache |
---|---|---|
dc.contributor.advisor | von Luck, Kai | - |
dc.contributor.author | Soblechero Salvado, André | - |
dc.date.accessioned | 2024-08-16T06:59:31Z | - |
dc.date.available | 2024-08-16T06:59:31Z | - |
dc.date.created | 2022-02-21 | - |
dc.date.issued | 2024-08-16 | - |
dc.identifier.uri | https://hdl.handle.net/20.500.12738/16167 | - |
dc.description.abstract | Machine learning is currently widely used for recommender systems. Previous work often represents users using unordered feature vectors while ignoring the sequential nature of users’ actions. In addition, classification models are often used which are limited by the time that they are trained. User history has grown exponentially since the age of Big Data, which implies the need to constantly retrain classification models. This thesis proposes a method which uses representations of users as time-series of embeddings, replacing inflexible standard feature vectors and considering the sequential nature of users’ actions. These user representations are then used to train a causal transformer decoder regression model which outputs the embedding of the next entry in the timeseries, making it unnecessary to constantly retrain the model. The A/B testing results show that the proposed method works. | en |
dc.description.abstract | Maschinelles Lernen wird heutzutage häufig in Empfehlungssystemen verwendet. Bisherige Arbeiten haben die Benutzer oftmals als nicht sortierte Feature-Vektoren dargestellt. Dies ignoriert die sequentielle Verhaltensweise eines Benutzers. Ebenfalls werden in vielen Fällen Klassifikationsmodelle verwendet, welche durch den Zeitpunkt des Trainings beschränkt sind. Seit Big Data steigt die Anzahl der Interaktionen von Benutzern exponentiell. Diese Tatsache impliziert, dass Klassifikationsmodelle konstant neu trainiert werden müssen. In dieser Arbeit wird eine Methode vorgestellt, die Benutzer als eine Zeitreihe von Einbettungen repräsentiert. Diese Zeitreihen ermöglichen es nicht flexible Feature-Vektoren zu ersetzen sowie die sequentielle Verhaltensweise eines Benutzers mit einzubeziehen. Die erwähnten Zeitreihen werden verwendet, um ein kausales Transformer Dekodierer Regressions Modell zu trainieren, welches die jeweils nächste Einbettung vorhersagt. Hierdurch wird verhindert, dass das Modell konstant neu trainiert werden muss. Die A/B-Tests demonstrieren, dass die vorgestellte Methode funktioniert. | de |
dc.language.iso | en | en_US |
dc.subject | Recommender System | en_US |
dc.subject | Natural Language Processing | en_US |
dc.subject | Next Click Prediction | en_US |
dc.subject | Sentence Embeddings | en_US |
dc.subject | Language Agnostic | en_US |
dc.subject.ddc | 004: Informatik | en_US |
dc.title | Language Agnostic News Recommendations Using Deep Learning Based Causal Transformer Decoder | en |
dc.type | Thesis | en_US |
openaire.rights | info:eu-repo/semantics/openAccess | en_US |
thesis.grantor.department | Fakultät Technik und Informatik | en_US |
thesis.grantor.department | Department Informatik | en_US |
thesis.grantor.universityOrInstitution | Hochschule für Angewandte Wissenschaften Hamburg | en_US |
tuhh.contributor.referee | Tiedemann, Tim | - |
tuhh.identifier.urn | urn:nbn:de:gbv:18302-reposit-191861 | - |
tuhh.oai.show | true | en_US |
tuhh.publication.institute | Fakultät Technik und Informatik | en_US |
tuhh.publication.institute | Department Informatik | en_US |
tuhh.type.opus | Masterarbeit | - |
dc.type.casrai | Supervised Student Publication | - |
dc.type.dini | masterThesis | - |
dc.type.driver | masterThesis | - |
dc.type.status | info:eu-repo/semantics/publishedVersion | en_US |
dc.type.thesis | masterThesis | en_US |
dcterms.DCMIType | Text | - |
tuhh.dnb.status | domain | en_US |
item.creatorGND | Soblechero Salvado, André | - |
item.grantfulltext | open | - |
item.openairetype | Thesis | - |
item.advisorGND | von Luck, Kai | - |
item.fulltext | With Fulltext | - |
item.languageiso639-1 | en | - |
item.cerifentitytype | Publications | - |
item.creatorOrcid | Soblechero Salvado, André | - |
item.openairecristype | http://purl.org/coar/resource_type/c_46ec | - |
Enthalten in den Sammlungen: | Theses |
Dateien zu dieser Ressource:
Datei | Beschreibung | Größe | Format | |
---|---|---|---|---|
MA_Language_Agnostic_News_REcommendations.pdf | 2.26 MB | Adobe PDF | Öffnen/Anzeigen |
Feedback zu diesem Datensatz
Export
Alle Ressourcen in diesem Repository sind urheberrechtlich geschützt.