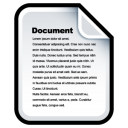
DC Element | Wert | Sprache |
---|---|---|
dc.contributor.advisor | von Luck, Kai | - |
dc.contributor.author | Kanne-Schludde, Thomas | - |
dc.date.accessioned | 2024-11-20T07:21:27Z | - |
dc.date.available | 2024-11-20T07:21:27Z | - |
dc.date.created | 2021-04-16 | - |
dc.date.issued | 2024-11-20 | - |
dc.identifier.uri | https://hdl.handle.net/20.500.12738/16540 | - |
dc.description.abstract | In dieser Arbeit wird eine „state-of-the-art“ Deep Learning Architektur trainiert, um die Kameraposition aus Fotos bekannter Objekte anhand der Pixel zu rekonstruieren. Der Ansatz wird als Klassifikationsproblem auf Basis synthetisch hergestellter Trainingsdaten definiert und anhand exemplarischer realer Testdaten die Machbarkeit evaluiert. Zudem erfolgt ein Vergleich mit der Verwendung realer Fotos als Trainingsgrundlage und weiterer Ansätze zur Bestimmung von Geopositionen. | de |
dc.description.abstract | In this work, a state-of-the-art Deep Learning architecture is trained to reconstruct the camera position from photographs of known objects based on the pixels. The approachis defined as a classification problem based on synthetically produced training data, and feasibility is evaluated using exemplary real test data. In addition, a comparison is made with the use of real photos as a training basis and other approaches for the determination of geo-positions. | en |
dc.language.iso | de | en_US |
dc.subject | Computer Vision | en_US |
dc.subject | Machine Learning | en_US |
dc.subject | Deep Learning | en_US |
dc.subject | neuronale Netze | en_US |
dc.subject | CNN | en_US |
dc.subject | Bilderkennung | en_US |
dc.subject | inhaltsbasierte Ortserkennung | en_US |
dc.subject | Geoposition | en_US |
dc.subject | Kameraposition | en_US |
dc.subject | GPS-Bestimmung | en_US |
dc.subject | Klassifikation | en_US |
dc.subject | neural networks | en_US |
dc.subject | image recognition | en_US |
dc.subject | content-based image retrieval | en_US |
dc.subject | visual place recognition | en_US |
dc.subject.ddc | 004: Informatik | en_US |
dc.title | Rekonstruktion der Kameraposition aus Fotos bekannter Objekte mit Deep Learning-Verfahren | de |
dc.type | Thesis | en_US |
openaire.rights | info:eu-repo/semantics/openAccess | en_US |
thesis.grantor.department | Department Informatik | en_US |
thesis.grantor.universityOrInstitution | Hochschule für Angewandte Wissenschaften Hamburg | en_US |
tuhh.contributor.referee | Meisel, Andreas | - |
tuhh.identifier.urn | urn:nbn:de:gbv:18302-reposit-197762 | - |
tuhh.oai.show | true | en_US |
tuhh.publication.institute | Department Informatik | en_US |
tuhh.publication.institute | Fakultät Technik und Informatik | en_US |
tuhh.type.opus | Masterarbeit | - |
dc.type.casrai | Supervised Student Publication | - |
dc.type.dini | masterThesis | - |
dc.type.driver | masterThesis | - |
dc.type.status | info:eu-repo/semantics/publishedVersion | en_US |
dc.type.thesis | masterThesis | en_US |
dcterms.DCMIType | Text | - |
tuhh.dnb.status | domain | en_US |
item.grantfulltext | open | - |
item.creatorGND | Kanne-Schludde, Thomas | - |
item.cerifentitytype | Publications | - |
item.creatorOrcid | Kanne-Schludde, Thomas | - |
item.advisorGND | von Luck, Kai | - |
item.languageiso639-1 | de | - |
item.openairecristype | http://purl.org/coar/resource_type/c_46ec | - |
item.fulltext | With Fulltext | - |
item.openairetype | Thesis | - |
Enthalten in den Sammlungen: | Theses |
Dateien zu dieser Ressource:
Datei | Beschreibung | Größe | Format | |
---|---|---|---|---|
MA_Rekonstruktion der Kameraposition aus Fotos bekannter Objekte mit Deep Learning-Verfahren_geschwärzt.pdf | 5.31 MB | Adobe PDF | Öffnen/Anzeigen |
Feedback zu diesem Datensatz
Export
Alle Ressourcen in diesem Repository sind urheberrechtlich geschützt.