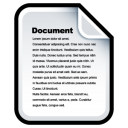
Title: | Interpretierbarkeit neuronaler Klassifikatoren mit Hilfe von Variational Autoencodern | Language: | German | Authors: | Grauer, Ilja | Keywords: | XAI; Variational Autoencoder; Interpretierbarkeit; Bildähnlichkeit; latenter Raum; ncc; interpretability; image similarity; latent space | Issue Date: | 5-Feb-2025 | Abstract: | Diese Arbeit kombiniert einen neuronalen Klassifikator mit einem Variational Autoencoder, um die Interpretierbarkeit ersteren zu verbessern. Die Repräsentation der Trainingsdaten im latenten Raum wird dafür genutzt die Ähnlichkeit zu einem Eingangsbild zu ermitteln. Normalized Cross Correlation wird als zusätzliche Vergleichsfunktion angewandt. Das Modell wird in verschiedenen Experimenten evaluiert und hinsichtlich seiner Relevanz diskutiert. Abschließend werden die offenen Probleme beschrieben und mögliche Lösungsansätze für zukünftige Arbeiten vorgeschlagen. This thesis combines a neural classifier with a variational autoencoder to improve the formers inter-pretability. The disentangled representation of the training data in the latent space is used to calculate the similarity to a sample image. Normalized cross correlation is used as a second similarity measurement. The model is evaluated in different experiments and discussed regarding its relevance. Finally, the unsolved problems are described and possible solutions for future works are suggested. |
URI: | https://hdl.handle.net/20.500.12738/16994 | Institute: | Department Informatik Fakultät Technik und Informatik |
Type: | Thesis | Thesis type: | Master Thesis | Advisor: | Meisel, Andreas | Referee: | Tiedemann, Tim |
Appears in Collections: | Theses |
Files in This Item:
File | Description | Size | Format | |
---|---|---|---|---|
MA_Interpretierbarkeit neuronaler Klassifikatoren mit Hilfe von Variational Autoencodern_geschwärzt.pdf | 8.12 MB | Adobe PDF | View/Open |
Note about this record
Export
Items in REPOSIT are protected by copyright, with all rights reserved, unless otherwise indicated.