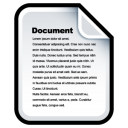
DC Field | Value | Language |
---|---|---|
dc.contributor.advisor | Putzar, Larissa | - |
dc.contributor.author | Wernst, Leander | - |
dc.date.accessioned | 2025-03-18T07:52:12Z | - |
dc.date.available | 2025-03-18T07:52:12Z | - |
dc.date.created | 2024-05-07 | - |
dc.date.issued | 2025-03-18 | - |
dc.identifier.uri | https://hdl.handle.net/20.500.12738/17313 | - |
dc.description.abstract | Deep Neural Networks (DNNs) benötigen zur effektiven Aufgabenbewältigung der Emotionserkennung anhand von Gesichtsausdrücken (Facial Expression Recognition [FER]) eine große Menge hochqualitativer Bilddaten. Dabei geht diese Arbeit davon aus, dass eine hohe Datenqualität mit einem hohen Detailgrad durch hohe Auflösungen einhergeht. Bestehende oder in unterschiedlichen Anwendungsszenarien generierte Daten können diesen Anforderungen oft nicht gerecht werden. Super-Resolution (SR) ist die Rekonstruktion hochaufgelöster Bilder aus niedrig aufgelösten und stellt einen vielversprechenden Ansatz zur Leistungssteigerung von FER durch Bild- und Auflösungsoptimierung dar, der in dieser Arbeit untersucht wird. Das Ziel der Analyse ist zu klären, welchen Effekt die Bildauflösung auf FER mit DNNs hat und inwiefern SR die Klassifikationsleistung durch Verbesserung degradierter Bilder beeinflussen kann. Hierzu werden mit EfficientNet-B0 und den FER-Datensätzen AffectNet und RaFD zunächst Versuche in unterschiedlichen durch Downsampling generierten Auflösungen durchgeführt. Anschließend werden die Bilder durch bikubische Interpolation sowie die SR-Verfahren HAT und GFPGAN upgesampelt und erneut getestet. Die höchste Accuracy des Auflösungsvergleichs wird jeweils bei 224 × 224 Pixeln mit 60,34 % (AffectNet, native Auflösung) bzw. 93,4 % (RaFD, downgesampelt) erzielt. Eine verbesserte Leistung durch das Upsampling niedriger aufgelöster Bilder durch SR gegenüber bikubischer Interpolation wird abgesehen von einem speziellen Fall nicht festgestellt. Die Ergebnisse deuten darauf hin, dass in SR Potenzial zur Leistungssteigerung von FER steckt, aber eine bessere Anpassung der SR-Modelle an die FER-Aufgabe vonnöten ist, um stärkere Verbesserungen zu bewirken. | de |
dc.description.abstract | Deep neural networks (DNNs) require a large amount of high-quality image data to effectively handle the task of facial expression recognition (FER). This work assumes that high data quality goes hand in hand with a high level of detail due to high resolutions. Existing data or data generated in various application scenarios often cannot meet these requirements. Super-Resolution (SR) is the reconstruction of high-resolution images from low-resolution images and represents a promising approach for increasing the performance of FER through image and resolution optimization, which is investigated in this thesis. The aim of the analysis is to study the effect of image resolution on FER with DNNs and to what extent SR can influence classification performance by improving degraded images. For this purpose, experiments with EfficientNet-B0 and the FER datasets AffectNet and RaFD are first performed at different resolutions generated by downsampling. The images are then upsampled using bicubic interpolation and the SR methods HAT and GFPGAN and tested again. The highest accuracy of the resolution comparison is achieved at 224 × 224 pixels with 60.34 % (AffectNet, native resolution) and 93.4 % (RaFD, downsampled). An improved performance by upsampling lower resolution images by SR compared to bicubic interpolation is not observed except for one specific case. The results indicate that SR has the potential to improve the performance of FER, but that SR models need to be adapted more closely to the FER task in order to achieve stronger improvements. | en |
dc.language.iso | de | en_US |
dc.subject.ddc | 004: Informatik | en_US |
dc.title | Super-Resolution in der Emotionserkennung : Effekte auflösungsoptimierter Bilder auf die Klassifikation von Gesichtsausdrücken durch Deep Neural Networks | de |
dc.type | Thesis | en_US |
openaire.rights | info:eu-repo/semantics/openAccess | en_US |
thesis.grantor.department | Fakultät Design, Medien und Information | en_US |
thesis.grantor.department | Department Medientechnik | en_US |
thesis.grantor.universityOrInstitution | Hochschule für Angewandte Wissenschaften Hamburg | en_US |
tuhh.contributor.referee | Ortmann, Thorben | - |
tuhh.identifier.urn | urn:nbn:de:gbv:18302-reposit-209262 | - |
tuhh.oai.show | true | en_US |
tuhh.publication.institute | Fakultät Design, Medien und Information | en_US |
tuhh.publication.institute | Department Medientechnik | en_US |
tuhh.type.opus | Bachelor Thesis | - |
dc.type.casrai | Supervised Student Publication | - |
dc.type.dini | bachelorThesis | - |
dc.type.driver | bachelorThesis | - |
dc.type.status | info:eu-repo/semantics/publishedVersion | en_US |
dc.type.thesis | bachelorThesis | en_US |
dcterms.DCMIType | Text | - |
tuhh.dnb.status | domain | en_US |
item.creatorGND | Wernst, Leander | - |
item.grantfulltext | open | - |
item.openairetype | Thesis | - |
item.advisorGND | Putzar, Larissa | - |
item.fulltext | With Fulltext | - |
item.languageiso639-1 | de | - |
item.cerifentitytype | Publications | - |
item.creatorOrcid | Wernst, Leander | - |
item.openairecristype | http://purl.org/coar/resource_type/c_46ec | - |
Appears in Collections: | Theses |
Files in This Item:
File | Description | Size | Format | |
---|---|---|---|---|
BA_Super-Resolution_Emotionserkennung.pdf | 16.94 MB | Adobe PDF | View/Open |
Note about this record
Export
Items in REPOSIT are protected by copyright, with all rights reserved, unless otherwise indicated.