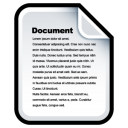
DC Field | Value | Language |
---|---|---|
dc.contributor.advisor | Hensel, Marc | - |
dc.contributor.author | Lanz, Finn | - |
dc.date.accessioned | 2025-03-19T07:59:12Z | - |
dc.date.available | 2025-03-19T07:59:12Z | - |
dc.date.created | 2023-07-11 | - |
dc.date.issued | 2025-03-19 | - |
dc.identifier.uri | https://hdl.handle.net/20.500.12738/17317 | - |
dc.description.abstract | In dieser Arbeit wird untersucht, wie neuronale Netzwerke trainiert werden können, damit diese Strategien zur Lösung von Zauberwürfeln entwickeln. Anschließend werden diese Netzwerke in geeignete Suchalgorithmen integriert, um die Effektivität und die Laufzeit bei der Lösungsfindung zu steigern. | de |
dc.description.abstract | This thesis investigates how neural networks can be trained to develop strategies for solving magic cubes. Subsequently, these networks are integrated into suitable search algorithms to enhance the effectiveness and efficiency of the solution finding process. | en |
dc.language.iso | de | en_US |
dc.subject | Maschinelles Lernen | en_US |
dc.subject | Neuronale Netze | en_US |
dc.subject | Deep Learning | en_US |
dc.subject | Reinforcement Learning | en_US |
dc.subject | Zauberwürfel | en_US |
dc.subject.ddc | 004: Informatik | en_US |
dc.title | Deep Reinforcement Learning zum maschinellen Erlernen von Strategien zur Lösung von Zauberwürfeln | de |
dc.type | Thesis | en_US |
openaire.rights | info:eu-repo/semantics/openAccess | en_US |
thesis.grantor.department | Fakultät Technik und Informatik | en_US |
thesis.grantor.department | Department Informations- und Elektrotechnik | en_US |
thesis.grantor.universityOrInstitution | Hochschule für Angewandte Wissenschaften Hamburg | en_US |
tuhh.contributor.referee | Appel, Sönke Christoph Wilhelm | - |
tuhh.identifier.urn | urn:nbn:de:gbv:18302-reposit-209344 | - |
tuhh.oai.show | true | en_US |
tuhh.publication.institute | Fakultät Technik und Informatik | en_US |
tuhh.publication.institute | Department Informations- und Elektrotechnik | en_US |
tuhh.type.opus | Masterarbeit | - |
dc.type.casrai | Supervised Student Publication | - |
dc.type.dini | masterThesis | - |
dc.type.driver | masterThesis | - |
dc.type.status | info:eu-repo/semantics/publishedVersion | en_US |
dc.type.thesis | masterThesis | en_US |
dcterms.DCMIType | Text | - |
tuhh.dnb.status | domain | en_US |
item.fulltext | With Fulltext | - |
item.openairetype | Thesis | - |
item.creatorOrcid | Lanz, Finn | - |
item.grantfulltext | open | - |
item.openairecristype | http://purl.org/coar/resource_type/c_46ec | - |
item.advisorGND | Hensel, Marc | - |
item.languageiso639-1 | de | - |
item.creatorGND | Lanz, Finn | - |
item.cerifentitytype | Publications | - |
Appears in Collections: | Theses |
Files in This Item:
File | Description | Size | Format | |
---|---|---|---|---|
MA_Deep_Reinforcement_Learning_maschinellen_Erlernen_Strategien.pdf | 7.79 MB | Adobe PDF | View/Open |
Note about this record
Export
Items in REPOSIT are protected by copyright, with all rights reserved, unless otherwise indicated.