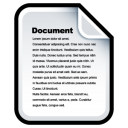
License: | ![]() |
Title: | Klassifikation hochvarianter Muster mit Faltungsnetzwerken | Language: | German | Authors: | Hassenklöver, Tobias | Issue Date: | 6-Mar-2012 | Abstract: | Die Klassifizierung von Objekten und besonders des Menschen durch Software stellt sich schon seit Jahren als schwer lösbares Problem heraus. Diese hochvarianten Formen werden optisch erfasst und bisher häufig mit Neuronalen Netzwerken in Kombination mit statistischen Verfahren erfasst. Eine neue Methode zur Erkennung von hochvarianten Mustern, wie Schriftzeichen, Gegenständen oder Menschen sind Faltungsnetzwerke. Faltungsnetzwerke sind eine Abart von Neuronalen Netzwerken, die ihre Entscheidungen auf Basis von Algorithmen aus der Bildverarbeitung treffen. In dieser Arbeit werden die Grenzen der Erkennung der Faltungsnetzwerke getestet. In Tests mit unterschiedlich modifizierten Eingabedaten wird die Auswirkung auf die Klassifizierungsgenauigkeit geprüft. The classification of objects and especially of humans by software is for years a great challenge. These highly variant forms are optically captured and often detected with the use of neural networks in combination with statistical methods. A new method for the detection of these highly variant patterns, such as characters, objects or people are Convolutional neural networks. Convolutional neural networks are a variety of Neural networks, which make their decisions based on algorithms used in image processing. In this thesis the limits of the detection of Convolutional neural networks are tested. In the tests the impact on the classification accuracy is checked with various modified input data. |
URI: | http://hdl.handle.net/20.500.12738/5702 | Institute: | Department Informatik | Type: | Thesis | Thesis type: | Bachelor Thesis | Advisor: | Meisel, Andreas | Referee: | Fohl, Wolfgang |
Appears in Collections: | Theses |
Files in This Item:
File | Description | Size | Format | |
---|---|---|---|---|
Bachelorarbeit.pdf | 5.76 MB | Adobe PDF | View/Open |
Note about this record
Export
Items in REPOSIT are protected by copyright, with all rights reserved, unless otherwise indicated.