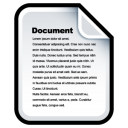
License: | ![]() |
Title: | Eignungsuntersuchung von Klassifikations- und Deep-Learning-Verfahren zurkamerabasierten Erkennung natürlicher Objekte | Other Titles: | Examination of classification and deep learning procedures for the camera basedrecognition of natural objects. | Language: | German | Authors: | Drössler, Lars | Keywords: | Convolutional Neural Network; SIFT; SURF; ORB; AKAZE; Deep-Learning; Food; Computer Vision; Haar-like Features; Convolutional Neural Network; SIFT; SURF; ORB; AKAZE; Deep Learning; Food; Computer Vision; Haar-like features | Issue Date: | 23-Jun-2017 | Abstract: | In dieser Arbeit wird eine Auswahl an Verfahren vorgestellt und auf ihre Eignung zur Klassifizierung natürlicher Objekte untersucht. Hierzu werden eine Reihe von Experimenten basierend auf publizierten Verfahren durchgeführt, in denen überprüft wird, wie gut die entsprechenden Verfahren natürliche Objekte in Fotos erkennen können. Als natürliche Objekte wurden für diese Testreihe verschiedene Gemüse- und Obstsorten gewählt. Diese Arbeit ist dazu ausgelegt, als Entscheidungshilfe für die Erstellung von Anwendungen zu dienen, in welchen es notwendig ist natürliche Objekte korrekt zu klassifizieren. This paper presents a selection of methods and examines them for their suitability to recognize natural objects. For this purpose, a series of experiments based on published procedures are conducted. These experiments test how said procedures perform and if they are used to recognize natural objects in images. The natural objects used in these experiments are different kinds of vegetables and fruits. This paper is intended to serve as a decision-making aid for the implementation of an application which should, amongst other things, recognize natural objects. |
URI: | http://hdl.handle.net/20.500.12738/8053 | Institute: | Department Informatik | Type: | Thesis | Thesis type: | Bachelor Thesis | Advisor: | von Luck, Kai | Referee: | Meisel, Andreas |
Appears in Collections: | Theses |
Files in This Item:
File | Description | Size | Format | |
---|---|---|---|---|
Bachelorarbeit_Lars_Droessler.pdf | 1.48 MB | Adobe PDF | View/Open |
Note about this record
Export
Items in REPOSIT are protected by copyright, with all rights reserved, unless otherwise indicated.