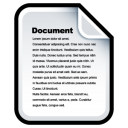
DC Element | Wert | Sprache |
---|---|---|
dc.contributor.advisor | von Luck, Kai | - |
dc.contributor.author | Assendorp, Jan Paul | |
dc.date.accessioned | 2020-09-29T14:25:46Z | - |
dc.date.available | 2020-09-29T14:25:46Z | - |
dc.date.created | 2017 | |
dc.date.issued | 2017-11-22 | |
dc.identifier.uri | http://hdl.handle.net/20.500.12738/8162 | - |
dc.description.abstract | Das Erkennen von Anomalien in Sensordaten ist ein wichtiger Anwendungsfall in der Industrie, um Fehler in maschinellen Prozessen frühzeitig erkennen zu können und potentiellen Schäden vorzubeugen. In dieser Arbeit wird ein Deep-Learning-Verfahren entwickelt, welches in mehrdimensionalen Sensordaten ungewöhnliche Muster erkennen kann. Dafür werden Echtdaten aus einer industriellen Anwendung verwendet. | de |
dc.description.abstract | Anomaly detection is crucial for the procactive detection of fatal failures of machines in industry applications. This thesis implements a deep learning algorithm for the task of anomaly detection in multivariate sensor data. The dataset is taken from a real-world application. | en |
dc.language.iso | de | de |
dc.rights.uri | http://rightsstatements.org/vocab/InC/1.0/ | - |
dc.subject | Deep-Learning | de |
dc.subject | Machine-Learning | de |
dc.subject.ddc | 004 Informatik | |
dc.title | Deep learning for anomaly detection in multivariate time series data | de |
dc.type | Thesis | |
openaire.rights | info:eu-repo/semantics/openAccess | |
thesis.grantor.department | Department Informatik | |
thesis.grantor.place | Hamburg | |
thesis.grantor.universityOrInstitution | Hochschule für angewandte Wissenschaften Hamburg | |
tuhh.contributor.referee | Meisel, Andreas | - |
tuhh.gvk.ppn | 1005248966 | |
tuhh.identifier.urn | urn:nbn:de:gbv:18302-reposit-81644 | - |
tuhh.note.extern | publ-mit-pod | |
tuhh.note.intern | 1 | |
tuhh.oai.show | true | en_US |
tuhh.opus.id | 4110 | |
tuhh.publication.institute | Department Informatik | |
tuhh.type.opus | Masterarbeit | - |
dc.subject.gnd | Anomalieerkennung | |
dc.type.casrai | Supervised Student Publication | - |
dc.type.dini | masterThesis | - |
dc.type.driver | masterThesis | - |
dc.type.status | info:eu-repo/semantics/publishedVersion | |
dc.type.thesis | masterThesis | |
dcterms.DCMIType | Text | - |
tuhh.dnb.status | domain | - |
item.grantfulltext | open | - |
item.creatorGND | Assendorp, Jan Paul | - |
item.cerifentitytype | Publications | - |
item.creatorOrcid | Assendorp, Jan Paul | - |
item.advisorGND | von Luck, Kai | - |
item.languageiso639-1 | de | - |
item.openairecristype | http://purl.org/coar/resource_type/c_46ec | - |
item.fulltext | With Fulltext | - |
item.openairetype | Thesis | - |
Enthalten in den Sammlungen: | Theses |
Dateien zu dieser Ressource:
Datei | Beschreibung | Größe | Format | |
---|---|---|---|---|
thesis_assendorp_2017.pdf | 3.03 MB | Adobe PDF | Öffnen/Anzeigen |
Feedback zu diesem Datensatz
Export
Alle Ressourcen in diesem Repository sind urheberrechtlich geschützt.