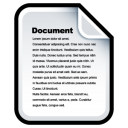
DC Field | Value | Language |
---|---|---|
dc.contributor.advisor | von Luck, Kai | - |
dc.contributor.author | Lühr, Lukas | |
dc.date.accessioned | 2020-09-29T14:30:08Z | - |
dc.date.available | 2020-09-29T14:30:08Z | - |
dc.date.created | 2017 | |
dc.date.issued | 2018-02-05 | |
dc.identifier.uri | http://hdl.handle.net/20.500.12738/8227 | - |
dc.description.abstract | In dieser Arbeit wird der Knowledge Discovery in Databases (KDD) Prozess exemplarisch anhand von Log les, welche im Rahmen eines biochemischen Fertigungsprozesses entstanden sind, durchlaufen. Diese Log les enthalten aus einfachen Daten aggregierte Strukturen, welche auch als Complex Objects bezeichnet werden. Es wird geprüft, inwieweit eine Bearbeitung der Complex Objects anhand des klassichen KDD Prozesses möglich ist. In dem Zuge werden auch verschiedene Ansätze des Maschinellen Lernens gegenübergestellt und bezüglich ihrer Leistungsfähigkeit auf den gegebenen Daten verglichen. Hierbei werden Entscheidungsbäume und verschiede Architekturen von neuronalen Netzen wie das Perzeptron, Multi Layer Perzeptron und Long short-term memory Netzwerke betrachtet. | de |
dc.description.abstract | In this work, the Knowledge Discovery in Databases (KDD) process is exemplarily executed on the basis of log les, which were created as part of a biochemical manufacturing process. These log les contain structures which are aggregated of simple data, these structures are called complex objects. It will be examined to what extent it is possible to apply the classic KDD process to these complex objects and what kind of result is to be expected. Therefor various approaches of machine learning are compared in terms of their performance on the given data. In particular, decision trees and some architectures of neural networks such as the perceptron, multi-layer perceptron and long short-term memory networks are considered. | en |
dc.language.iso | de | de |
dc.rights.uri | http://rightsstatements.org/vocab/InC/1.0/ | - |
dc.subject.ddc | 004 Informatik | |
dc.title | Eignung von maschinellen Lernverfahren im Kontext eines KDD Prozesses für biochemische Prozessdaten | de |
dc.type | Thesis | |
openaire.rights | info:eu-repo/semantics/openAccess | |
thesis.grantor.department | Department Informatik | |
thesis.grantor.place | Hamburg | |
thesis.grantor.universityOrInstitution | Hochschule für angewandte Wissenschaften Hamburg | |
tuhh.contributor.referee | Tiedemann, Tim | - |
tuhh.gvk.ppn | 1012330966 | |
tuhh.identifier.urn | urn:nbn:de:gbv:18302-reposit-82294 | - |
tuhh.note.extern | publ-mit-pod | |
tuhh.note.intern | 1 | |
tuhh.oai.show | true | en_US |
tuhh.opus.id | 4172 | |
tuhh.publication.institute | Department Informatik | |
tuhh.type.opus | Bachelor Thesis | - |
dc.subject.gnd | Maschinelles Lernen | |
dc.type.casrai | Supervised Student Publication | - |
dc.type.dini | bachelorThesis | - |
dc.type.driver | bachelorThesis | - |
dc.type.status | info:eu-repo/semantics/publishedVersion | |
dc.type.thesis | bachelorThesis | |
dcterms.DCMIType | Text | - |
tuhh.dnb.status | domain | - |
item.advisorGND | von Luck, Kai | - |
item.languageiso639-1 | de | - |
item.fulltext | With Fulltext | - |
item.creatorGND | Lühr, Lukas | - |
item.openairetype | Thesis | - |
item.grantfulltext | open | - |
item.creatorOrcid | Lühr, Lukas | - |
item.cerifentitytype | Publications | - |
item.openairecristype | http://purl.org/coar/resource_type/c_46ec | - |
Appears in Collections: | Theses |
Files in This Item:
File | Description | Size | Format | |
---|---|---|---|---|
Bachelorarbeit_Lukas_Luehr.pdf | 1.34 MB | Adobe PDF | View/Open |
Note about this record
Export
Items in REPOSIT are protected by copyright, with all rights reserved, unless otherwise indicated.