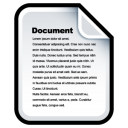
DC Field | Value | Language |
---|---|---|
dc.contributor.advisor | Zukunft, Olaf | - |
dc.contributor.author | Kamp, Birger | |
dc.date.accessioned | 2020-09-29T14:37:01Z | - |
dc.date.available | 2020-09-29T14:37:01Z | - |
dc.date.created | 2018 | |
dc.date.issued | 2018-05-29 | |
dc.identifier.uri | http://hdl.handle.net/20.500.12738/8318 | - |
dc.description.abstract | Der Bereich der Datenintegration ist auf Prozesse und Tools angewiesen, die Daten effektiv und effzient verarbeiten. Viele Tools und Frameworks, die für Big Data-Problemstellungen entwickelt werden, eignen sich auch für die Nutzung im Datenintegrationsbereich. In dieser Arbeit werden ein bekanntes ETL-Tool und eine Kombination aus zwei Big Data-Frameworks miteinander verglichen. Die TPC, ein Non-Profit-Konsortium aus Datenintegrationsherstellern, hat den ETL-Benchmark TPC-DI spezifiziert, durch welchen ETL-Tools und -Frameworks miteinander verglichen werden können. Die komplexe Spezifikation wird mit dem Tool Talend Open Studio for Big Data und einer Kombination aus den Big Data-Frameworks Apache Gobblin und Apache Spark implementiert. Einer der Importjobs wird außerdem so modifiziert, dass er einen Event-Stream statt eines Dateisystems als Datenquelle verwendet. Zur Zeit des Schreibens ist diese Arbeit die einzige Veröffentlichung, die den TPC-DI-Benchmark mit diesen beiden Messkandidaten und der Streaming-Erweiterung durchführt. Die Messungen werden in mehr als 50 unterschiedlichen Experimenten durchgeführt. Dadurch werden viele Unterschiede der Implementationen, sowohl in den Importlaufzeiten, als auch in der CPU- und RAM-Nutzung, sichtbar. Die Ergebnisse zeigen, dass die Talend-Implementation mehr CPU-Last auf dem Datenbankserver erzeugt als die Gobblin-Spark-Implementation. Während die Gobblin-Spark-Implementation mehr CPU-Last auf den ausführenden Nodes ausführt als die Talend-Implementation. In den Laufzeiten ist zu sehen, dass bei den zwei kleinsten Importdatensets, die Gobblin-Spark-Implementation die kürzeste Laufzeit erreicht. Im größten gemessenen Datenset hingegen erzielt Talend die geringere Laufzeit. | de |
dc.description.abstract | The field of data integration relies on processes and tools that process data effectively and effciently. Many tools and frameworks that are developed for big data problems are also suitable for use in data integration. This paper compares a well-known ETL tool and a combination of two big data frameworks. The TPC, a non-profit consortium of data integration vendors, has specified the ETL benchmark TPC-DI, which compares ETL tools and frameworks. The extensive specification is implemented with the tool Talend Open Studio for Big Data and a combination of the Big Data frameworks Apache Gobblin and Apache Spark. One of the import jobs is also modified to use an event stream instead of a file system as the data source. At the time of writing, this work is the only publication that performs the TPC-DI benchmark with these two candidate candidates and a streaming extension. The measurements are carried out in more than 50 different experiments. As a result, many dfferences in the implementation, both in the import runtime, as well as in the CPU and RAM usage, will be visible. The results show that the Talend implementation generates more CPU load on the database server than the Gobblin Spark implementation. While the Gobblin Spark implementation runs more CPU on the executing nodes than the Talend implementation. In terms of runtime, the Gobblin Spark implementation achieves the shortest runtime for the two smallest import data sets. By contrast, Talend achieves the lower running time in the largest measured data set. | en |
dc.language.iso | de | de |
dc.rights.uri | http://rightsstatements.org/vocab/InC/1.0/ | - |
dc.subject.ddc | 004 Informatik | |
dc.title | Evaluation von Open Source Data Integration Tools im Kontext von Big Data | de |
dc.type | Thesis | |
openaire.rights | info:eu-repo/semantics/openAccess | |
thesis.grantor.department | Department Informatik | |
thesis.grantor.place | Hamburg | |
thesis.grantor.universityOrInstitution | Hochschule für angewandte Wissenschaften Hamburg | |
tuhh.contributor.referee | Steffens, Ulrike | - |
tuhh.gvk.ppn | 1023389177 | |
tuhh.identifier.urn | urn:nbn:de:gbv:18302-reposit-83200 | - |
tuhh.note.extern | publ-mit-pod | |
tuhh.note.intern | 1 | |
tuhh.oai.show | true | en_US |
tuhh.opus.id | 4256 | |
tuhh.publication.institute | Department Informatik | |
tuhh.type.opus | Masterarbeit | - |
dc.subject.gnd | Massendaten | |
dc.type.casrai | Supervised Student Publication | - |
dc.type.dini | masterThesis | - |
dc.type.driver | masterThesis | - |
dc.type.status | info:eu-repo/semantics/publishedVersion | |
dc.type.thesis | masterThesis | |
dcterms.DCMIType | Text | - |
tuhh.dnb.status | domain | - |
item.creatorGND | Kamp, Birger | - |
item.fulltext | With Fulltext | - |
item.creatorOrcid | Kamp, Birger | - |
item.grantfulltext | open | - |
item.cerifentitytype | Publications | - |
item.advisorGND | Zukunft, Olaf | - |
item.languageiso639-1 | de | - |
item.openairecristype | http://purl.org/coar/resource_type/c_46ec | - |
item.openairetype | Thesis | - |
Appears in Collections: | Theses |
Files in This Item:
File | Description | Size | Format | |
---|---|---|---|---|
Masterthesis_Kamp.pdf | 14.73 MB | Adobe PDF | View/Open |
Note about this record
Export
Items in REPOSIT are protected by copyright, with all rights reserved, unless otherwise indicated.