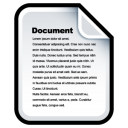
DC Field | Value | Language |
---|---|---|
dc.contributor.advisor | von Luck, Kai | - |
dc.contributor.author | Anders, Lucas | |
dc.date.accessioned | 2020-09-29T14:51:41Z | - |
dc.date.available | 2020-09-29T14:51:41Z | - |
dc.date.created | 2018 | |
dc.date.issued | 2018-12-14 | |
dc.identifier.uri | http://hdl.handle.net/20.500.12738/8528 | - |
dc.description.abstract | Die kurzfristige Prognose des Energiebedarfs ist für Energieversorger ein interessanter Anwendungsfall und kritischer Bestandteil eines effizienten Energiemanagementsystems. In dieser Arbeit wird ein intelligentes Vorhersagemodell entwickelt, welches den Gesamtenergiebedarf eines abgelegenen Forschungsgebäudes auf der Basis von realen und multidimensionalen Sensordaten prognostizieren kann. Die verwendeten Daten entstammen der Polarforschungsstation Neumayer III. Dabei werden verschiedene zeitreihenbasierte Deep-Learning-Verfahren eingesetzt und evaluiert. | de |
dc.description.abstract | Predicting the short-term energy consumption is an interesting use case for energy providers and crucial for an efficient energy management system. This thesis implements an intelligent model which predicts short-term energy demands based on real and multivariate sensor data. The dataset is taken from the antarctic research facility Neumayer III. Different deep learning models for time series prediction are applied and evaluated. | en |
dc.language.iso | de | de |
dc.rights.uri | http://rightsstatements.org/vocab/InC/1.0/ | - |
dc.subject | Deep-Learning | de |
dc.subject | Machine-Learning | de |
dc.subject | Zeitreihendaten | de |
dc.subject | Sensordaten | de |
dc.subject | Long-Short-Term-Memory-Networks | de |
dc.subject | Deep learning | en |
dc.subject | Machine Learning | en |
dc.subject | Time-Series Data | en |
dc.subject | Sensor Data | en |
dc.subject | Long-Short-Term-Memory-Networks | en |
dc.subject.ddc | 004 Informatik | |
dc.title | Deep Learning zur Vorhersage des Energiebedarfs der antarktischen Forschungsstation Neumayer III | de |
dc.title.alternative | Deep Learning for energy consumption prediction of the antarctic research facility Neumayer III | en |
dc.type | Thesis | |
openaire.rights | info:eu-repo/semantics/openAccess | |
thesis.grantor.department | Department Informatik | |
thesis.grantor.place | Hamburg | |
thesis.grantor.universityOrInstitution | Hochschule für angewandte Wissenschaften Hamburg | |
tuhh.contributor.referee | Tiedemann, Tim | - |
tuhh.gvk.ppn | 1043290966 | |
tuhh.identifier.urn | urn:nbn:de:gbv:18302-reposit-85306 | - |
tuhh.note.extern | publ-mit-pod | |
tuhh.note.intern | 1 | |
tuhh.oai.show | true | en_US |
tuhh.opus.id | 4457 | |
tuhh.publication.institute | Department Informatik | |
tuhh.type.opus | Bachelor Thesis | - |
dc.subject.gnd | Deep learning | |
dc.subject.gnd | Maschinelles Lernen | |
dc.type.casrai | Supervised Student Publication | - |
dc.type.dini | bachelorThesis | - |
dc.type.driver | bachelorThesis | - |
dc.type.status | info:eu-repo/semantics/publishedVersion | |
dc.type.thesis | bachelorThesis | |
dcterms.DCMIType | Text | - |
tuhh.dnb.status | domain | - |
item.creatorGND | Anders, Lucas | - |
item.openairetype | Thesis | - |
item.openairecristype | http://purl.org/coar/resource_type/c_46ec | - |
item.creatorOrcid | Anders, Lucas | - |
item.languageiso639-1 | de | - |
item.grantfulltext | open | - |
item.cerifentitytype | Publications | - |
item.advisorGND | von Luck, Kai | - |
item.fulltext | With Fulltext | - |
Appears in Collections: | Theses |
Files in This Item:
File | Description | Size | Format | |
---|---|---|---|---|
bachelor_thesis_anders.pdf | 1.75 MB | Adobe PDF | View/Open |
Note about this record
Export
Items in REPOSIT are protected by copyright, with all rights reserved, unless otherwise indicated.