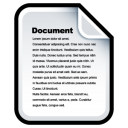
DC Field | Value | Language |
---|---|---|
dc.contributor.advisor | Neitzke, Michael | - |
dc.contributor.author | Gandraß, Niels | |
dc.date.accessioned | 2020-09-29T15:07:38Z | - |
dc.date.available | 2020-09-29T15:07:38Z | - |
dc.date.created | 2019 | |
dc.date.issued | 2019-05-28 | |
dc.identifier.uri | http://hdl.handle.net/20.500.12738/8767 | - |
dc.description.abstract | In dieser Bachelorarbeit wird ein Verfahren zur unüberwachten Merkmalsextraktion aus Aerosol-Rückstreuprofilen vorgestellt. Hierbei werden LIDAR-Ceilometer Messdatensätze zuerst einer Vorverarbeitung unterzogen, um dann mithilfe eines Convolutional-Autoencoders in komprimierte Merkmalsvektoren überführt zu werden. Auf Basis dieser Merkmalsvektoren findet ein initiales Clustering statt, welches durch Minimierung der KL-Divergenz zu einer gewählten Zielverteilung weiter optimiert wird. Zwei Experimente, zunächst auf dem MNIST-Zifferndatensatz und im Anschluss mit realen Ceilometerdaten, haben die Funktionsfähigkeit des Ansatzes bestätigt. Erfolgreich konnten so einige meteorologische Phänomene in Clustern gruppiert und halbautomatisch mit Labels versehen werden. Neben den Implementationsdetails des Verfahrens werden außerdem einige Eigenschaften der vorliegenden Messdaten besprochen sowie ein umfangreicher Ausblick auf weitere Forschung in diesem Gebiet gegeben. | de |
dc.description.abstract | In this bachelor thesis a method for unsupervised feature extraction from aerosol backscatter profiles is proposed. After a first data preprocessing step the LIDAR-ceilometer datasets are converted into an compressed representation using an convolutional autoencoder. These encoded representations are then used for the computation of an initial cluster assignment which gets further optimized, trough minimizing the KL-divergence to an arbitrary target distribution, during consecutive training. Two experiments, the first based on the MNIST database of handwritten digits and the second based on real ceilometer aerosol backscatter data, were successfully executed. The proposed method has been successfully utilized, in order to group some meteorological phenomena into clusters which then labels were assigned to. In addition to the description of implementation details, multiple aspects of the ceilometer datasets are analyzed and a comprehensive outlook to further possible research in this field is given. | en |
dc.language.iso | de | de |
dc.rights.uri | http://rightsstatements.org/vocab/InC/1.0/ | - |
dc.subject.ddc | 004 Informatik | |
dc.title | Merkmalsextraktion durch Methoden des unüberwachten maschinellen Lernens zur Klassifikation von Aerosol-Rückstreuprofilen aus LIDAR-Ceilometern | de |
dc.title.alternative | Unsupervised Machine Learning: Feature Extraction for Classification of LIDAR-Ceilometer Aerosol-Backscatter Profiles | en |
dc.type | Thesis | |
openaire.rights | info:eu-repo/semantics/openAccess | |
thesis.grantor.department | Department Informatik | |
thesis.grantor.place | Hamburg | |
thesis.grantor.universityOrInstitution | Hochschule für angewandte Wissenschaften Hamburg | |
tuhh.contributor.referee | Meisel, Andreas | - |
tuhh.gvk.ppn | 1666382442 | |
tuhh.identifier.urn | urn:nbn:de:gbv:18302-reposit-87693 | - |
tuhh.note.extern | publ-mit-pod | |
tuhh.note.intern | 1 | |
tuhh.oai.show | true | en_US |
tuhh.opus.id | 4870 | |
tuhh.publication.institute | Department Informatik | |
tuhh.type.opus | Bachelor Thesis | - |
dc.subject.gnd | Cluster | |
dc.type.casrai | Supervised Student Publication | - |
dc.type.dini | bachelorThesis | - |
dc.type.driver | bachelorThesis | - |
dc.type.status | info:eu-repo/semantics/publishedVersion | |
dc.type.thesis | bachelorThesis | |
dcterms.DCMIType | Text | - |
tuhh.dnb.status | domain | - |
item.creatorGND | Gandraß, Niels | - |
item.fulltext | With Fulltext | - |
item.creatorOrcid | Gandraß, Niels | - |
item.grantfulltext | open | - |
item.cerifentitytype | Publications | - |
item.advisorGND | Neitzke, Michael | - |
item.languageiso639-1 | de | - |
item.openairecristype | http://purl.org/coar/resource_type/c_46ec | - |
item.openairetype | Thesis | - |
Appears in Collections: | Theses |
Files in This Item:
File | Description | Size | Format | |
---|---|---|---|---|
Gandrass_2019_Merkmalsextraktion_Aerosol_Rueckstreuprofile_Ceilometer.pdf | 7.3 MB | Adobe PDF | View/Open |
Note about this record
Export
Items in REPOSIT are protected by copyright, with all rights reserved, unless otherwise indicated.