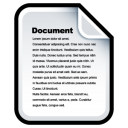
DC Field | Value | Language |
---|---|---|
dc.contributor.advisor | Jünemann, Klaus | - |
dc.contributor.author | Son, Jeonghyun | |
dc.date.accessioned | 2020-09-29T15:34:10Z | - |
dc.date.available | 2020-09-29T15:34:10Z | - |
dc.date.created | 2019 | |
dc.date.issued | 2019-11-08 | |
dc.identifier.uri | http://hdl.handle.net/20.500.12738/9168 | - |
dc.description.abstract | One of the limitations of supervised learning in deep learning algorithm is to gather and label a large set of data. In this document, the approach to solve this limitation is presented by using synthetic data. A scene of a real-like traffic situation with bicycles is created with 3D framework, THREE.js. The synthetic data is automatically generated with labels by taking a screenshot of rendering scene. The data is used to train on convolutional nerual network for image classification. At the end, the performance of convolutional neural network model is evaluated on real image dataset. | en |
dc.description.abstract | Eine große Herausforderung während des Trainings von faltenden neuronalen Netzwerken besteht darin, eine große Menge gekennzeichneter Daten zu Verfügung zu stellen. In dieser Arbeit wird ein Ansatz zur Lösung dieser Einschränkung durch die Verwendung synthetischer Trainingsdaten vorgestellt. Mithilfe des 3D Framework THREE.js wird eine Szene einer realitätsnahen Verkehrssituation mit Fahrrädern erzeugt. Anschließend werden synthetische Trainingsdaten mit Kennzeichnungen generiert, indem eine Vielzahl von Momentaufnahmen der Szene erstellt werden. Diese Daten werden anschließend verwendet, um ein faltendes neuronales Netzwerk für eine Bildklassifizierungsaufgabe zu trainieren. Abschließend wird die Leistung des Modells des Netzwerks mit Hilfe von realen Bilddaten bewertet. | de |
dc.language.iso | en | en |
dc.rights.uri | http://rightsstatements.org/vocab/InC/1.0/ | - |
dc.subject.ddc | 004 Informatik | |
dc.title | Evaluation of Convolutional Neural Network performance using synthetic data fortraining | en |
dc.type | Thesis | |
openaire.rights | info:eu-repo/semantics/openAccess | |
thesis.grantor.department | Department Informatik | |
thesis.grantor.place | Hamburg | |
thesis.grantor.universityOrInstitution | Hochschule für angewandte Wissenschaften Hamburg | |
tuhh.contributor.referee | Leutelt, Lutz | - |
tuhh.gvk.ppn | 168152063X | |
tuhh.identifier.urn | urn:nbn:de:gbv:18302-reposit-91706 | - |
tuhh.note.extern | publ-mit-pod | |
tuhh.note.intern | 1 | |
tuhh.oai.show | true | en_US |
tuhh.opus.id | 5254 | |
tuhh.publication.institute | Department Informatik | |
tuhh.type.opus | Bachelor Thesis | - |
dc.subject.gnd | Computergrafik | |
dc.subject.gnd | Maschinelles Lernen | |
dc.subject.gnd | Deep learning | |
dc.type.casrai | Supervised Student Publication | - |
dc.type.dini | bachelorThesis | - |
dc.type.driver | bachelorThesis | - |
dc.type.status | info:eu-repo/semantics/publishedVersion | |
dc.type.thesis | bachelorThesis | |
dcterms.DCMIType | Text | - |
tuhh.dnb.status | domain | - |
item.grantfulltext | open | - |
item.creatorGND | Son, Jeonghyun | - |
item.cerifentitytype | Publications | - |
item.creatorOrcid | Son, Jeonghyun | - |
item.advisorGND | Jünemann, Klaus | - |
item.languageiso639-1 | en | - |
item.openairecristype | http://purl.org/coar/resource_type/c_46ec | - |
item.fulltext | With Fulltext | - |
item.openairetype | Thesis | - |
Appears in Collections: | Theses |
Files in This Item:
File | Description | Size | Format | |
---|---|---|---|---|
Bachelorthesis_JeonghyunSon.pdf | 1.45 MB | Adobe PDF | View/Open |
Note about this record
Export
Items in REPOSIT are protected by copyright, with all rights reserved, unless otherwise indicated.