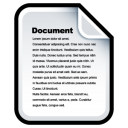
DC Field | Value | Language |
---|---|---|
dc.contributor.advisor | Neitzke, Michael | - |
dc.contributor.author | Kessener, Daniel | |
dc.date.accessioned | 2020-09-29T15:41:06Z | - |
dc.date.available | 2020-09-29T15:41:06Z | - |
dc.date.created | 2019 | |
dc.date.issued | 2020-01-13 | |
dc.identifier.uri | http://hdl.handle.net/20.500.12738/9274 | - |
dc.description.abstract | In dieser Arbeit wird die Eignung verschiedener Genetischer Algorithmen der NEAT-Familie für die Optimierung rekurrenter neuronaler Netze untersucht. Dabei werden konkret klassisches NEAT und ES-HyperNEAT in Augenschein genommen. Beide GAs werden mit verschiedenen RNN-Architekturen kombiniert. Konkret werden Long Short-Term Memory und Gated Recurrent Units with Memory Block verwendet. Es werden drei Untersuchungen mit verschiedenen Komplexitätsgraden und Ansprüche an das Erinnerungsvermögen der Agenten durchgeführt, die zeigen, dass GAs sich grundsätzlich gut zum Optimieren von RNNs eignen. | de |
dc.description.abstract | This paper tests how suited different Genetic Algorithms from the NEAT-family of GAs are to optimize recurrent artificial neural networks. Specifically this paper looks at classic NEAT and ES-HyperNEAT. Both GAs are combined with different RNN architectures - specifically Long Short-Term Memory and Gated Recurrent Units with Memory Block - and subjected to three tests of varying complexities and demands on the memory of the agents. It can be shown that GAs are able to optimize RNN reasonably well. | en |
dc.language.iso | de | de |
dc.rights.uri | http://rightsstatements.org/vocab/InC/1.0/ | - |
dc.subject.ddc | 004 Informatik | |
dc.title | Optimierung rekurrenter neuronaler Netze durch genetische Algorithmen derNEAT-Familie | de |
dc.type | Thesis | |
openaire.rights | info:eu-repo/semantics/openAccess | |
thesis.grantor.department | Department Informatik | |
thesis.grantor.place | Hamburg | |
thesis.grantor.universityOrInstitution | Hochschule für angewandte Wissenschaften Hamburg | |
tuhh.contributor.referee | Pareigis, Stephan | - |
tuhh.gvk.ppn | 1687213984 | |
tuhh.identifier.urn | urn:nbn:de:gbv:18302-reposit-92762 | - |
tuhh.note.extern | publ-mit-pod | |
tuhh.note.intern | 1 | |
tuhh.oai.show | true | en_US |
tuhh.opus.id | 5351 | |
tuhh.publication.institute | Department Informatik | |
tuhh.type.opus | Bachelor Thesis | - |
dc.subject.gnd | Algorithmus | |
dc.type.casrai | Supervised Student Publication | - |
dc.type.dini | bachelorThesis | - |
dc.type.driver | bachelorThesis | - |
dc.type.status | info:eu-repo/semantics/publishedVersion | |
dc.type.thesis | bachelorThesis | |
dcterms.DCMIType | Text | - |
tuhh.dnb.status | domain | - |
item.grantfulltext | open | - |
item.creatorGND | Kessener, Daniel | - |
item.cerifentitytype | Publications | - |
item.creatorOrcid | Kessener, Daniel | - |
item.advisorGND | Neitzke, Michael | - |
item.languageiso639-1 | de | - |
item.openairecristype | http://purl.org/coar/resource_type/c_46ec | - |
item.fulltext | With Fulltext | - |
item.openairetype | Thesis | - |
Appears in Collections: | Theses |
Files in This Item:
File | Description | Size | Format | |
---|---|---|---|---|
thesis.pdf | 1.53 MB | Adobe PDF | View/Open |
Note about this record
Export
Items in REPOSIT are protected by copyright, with all rights reserved, unless otherwise indicated.