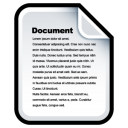
License: | ![]() |
Title: | Inertiale Navigation mittels Deep-Learning | Language: | German | Authors: | Meyer, Manuel | Keywords: | inertiale Navigation; INS; Trägheitsnavigation; künstliche Intelligenz; Machine Learning | Issue Date: | 28-Feb-2022 | Abstract: | Inertialsensoren spielen in der inertialen Navigation eine zentrale Rolle. Die Anforderungen die gängige inertiale Navigationssysteme mit sich bringen, können oftmals nicht alleine durch die Verwendung eines einzelnen Systems gestemmt werden, weshalb heutzutage vielfach auf integrierte Navigationssysteme gesetzt wird. Diese bringen aber einen hohen Implementierungs-, Wartungs- und Kostenaufwand mit sich. Allerdings hat die Entwicklung der letzten Jahre dazu geführt, dass sich kostengünstige Inertialsensoren in zahlreichen Bereichen und Anwendungen etabliert haben. Die kostengünstigen MEMS-Sensoren haben jedoch den großen Nachteil, dass sie mit der Zeit einen zunehmenden Schleppfehler verursachen, weshalb sie sich nur mit einem entsprechenden Referenzsystem (z. B. GNSS) zur Positionsbestimmung eignen. Ein Ansatz, mit dem es ermöglicht wird, auch den kostengünstigen Inertialsensoren wie sie mittlerweile in jedem Smartphone zu finden sind, eine Positionbestimmung zu erlauben, wäre daher wünschenswert. Gegenstand dieser Arbeit ist aus diesem Grund die Vorstellung eines neuartigen Lösungsansatzes einer Positionsbestimmung mittels Low-cost-Inertialsensoren auf Basis von tiefen neuronalen Netzen. Der Ansatz soll dabei umgebungsunabhängig und ohne ein entsprechendes Referenzsystem die Anforderungen der Zuverlässigkeit, Verfügbarkeit und Genauigkeit erfüllen können, sodass eine inertiale Navigation auch in abgeschatteten Bereichen wie der Indoor-Navigation garantiert werden kann. Inertial sensors play a major role in inertial navigation. The requirements of current inertial navigation systems often can not be solved by the use of a single system, which is the reason why integrated navigation systems are often used nowadays. But these systems involve high implementation, maintenance and expenditures. However, the development in recent years has led to the establishment of low-cost inertial sensors in various fields and applications. The low-cost MEMS sensors, however, have the big disadvantage that they cause unavoidable errors to the entire system over time, so that they are only suitable with a corresponding reference system (e.g. GNSS) for position determination. An approach that makes it possible to allow position determination even with low-cost inertial sensors as they are now to be found in any smartphone, would therefore be desirable. For this reason, the subject of this thesis is the presentation of a novel solution of position determination by means of low-cost inertial sensors based on deep neural networks. With this approach it should be possible to meet the requirements of reliability, availability and accuracy regardless of the environment and without a corresponding reference system, so that inertial navigation can also be guaranteed in shadowed areas such as indoor navigation. |
URI: | http://hdl.handle.net/20.500.12738/12530 | Institute: | Fakultät Technik und Informatik Department Informatik |
Type: | Thesis | Thesis type: | Master Thesis | Advisor: | Meisel, Andreas | Referee: | Fohl, Wolfgang |
Appears in Collections: | Theses |
Files in This Item:
File | Description | Size | Format | |
---|---|---|---|---|
MA_MeyerManuel_InertialeNavigationMittelsDeepLearning.pdf | 12.79 MB | Adobe PDF | View/Open |
Note about this record
Export
Items in REPOSIT are protected by copyright, with all rights reserved, unless otherwise indicated.