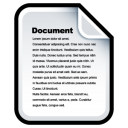
DC Field | Value | Language |
---|---|---|
dc.contributor.advisor | von Luck, Kai | - |
dc.contributor.author | Kamenz, Andreas | - |
dc.date.accessioned | 2022-03-01T08:38:20Z | - |
dc.date.available | 2022-03-01T08:38:20Z | - |
dc.date.created | 2019-04-29 | - |
dc.date.issued | 2022-03-01 | - |
dc.identifier.uri | http://hdl.handle.net/20.500.12738/12541 | - |
dc.description.abstract | A good emotion recognition is of great importance for companion systems in order to be able to respond to the user in the best possible way. In this master thesis the test setup and the laboratory study will be described. We propose serveral machine and deep learning methods to solve this problem. These methods will be implemented and compared to show that they contribute to an improved emotion recognition performance. | en_US |
dc.description.abstract | Eine gute Emotionserkennung ist für Companion Systeme von großer Bedeutung, um bestmöglich auf den Benutzer antworten zu können. In dieser Masterarbeit wird der erstellte Versuchsaufbau und der durchgeführte Laborversuch im Kontext Emotionserkennung beschrieben. Mehrere Machine- und Deep-Learning Verfahren werden vorgeschlagen, um dieses Problem zu lösen. Diese werden implementiert und miteinander verglichen, um einen Beitrag zu besseren Ergebnissen bei der Emotionserkennung zu leisten. | de |
dc.language.iso | en | en_US |
dc.rights.uri | http://rightsstatements.org/vocab/InC/1.0/ | - |
dc.subject | Deep Learning | en_US |
dc.subject | Machine Learning | en_US |
dc.subject | Companion Technology | en_US |
dc.subject | Affective Computing | en_US |
dc.subject.ddc | 004: Informatik | en_US |
dc.title | Time Series Analysis of Physiological Data for Emotion Recognition using Deep Learning | en_US |
dc.type | Thesis | en_US |
openaire.rights | info:eu-repo/semantics/openAccess | en_US |
thesis.grantor.department | Fakultät Technik und Informatik | en_US |
thesis.grantor.department | Department Informatik | en_US |
thesis.grantor.universityOrInstitution | Hochschule für Angewandte Wissenschaften Hamburg | en_US |
tuhh.contributor.referee | Tiedemann, Tim | - |
tuhh.identifier.urn | urn:nbn:de:gbv:18302-reposit-141532 | - |
tuhh.oai.show | true | en_US |
tuhh.publication.institute | Fakultät Technik und Informatik | en_US |
tuhh.publication.institute | Department Informatik | en_US |
tuhh.type.opus | Masterarbeit | - |
dc.type.casrai | Supervised Student Publication | - |
dc.type.dini | masterThesis | - |
dc.type.driver | masterThesis | - |
dc.type.status | info:eu-repo/semantics/publishedVersion | en_US |
dc.type.thesis | masterThesis | en_US |
dcterms.DCMIType | Text | - |
tuhh.dnb.status | domain | - |
item.creatorGND | Kamenz, Andreas | - |
item.fulltext | With Fulltext | - |
item.creatorOrcid | Kamenz, Andreas | - |
item.grantfulltext | open | - |
item.cerifentitytype | Publications | - |
item.advisorGND | von Luck, Kai | - |
item.languageiso639-1 | en | - |
item.openairecristype | http://purl.org/coar/resource_type/c_46ec | - |
item.openairetype | Thesis | - |
Appears in Collections: | Theses |
Files in This Item:
File | Description | Size | Format | |
---|---|---|---|---|
Masterarbeit.pdf | 11.5 MB | Adobe PDF | View/Open |
Note about this record
Export
Items in REPOSIT are protected by copyright, with all rights reserved, unless otherwise indicated.