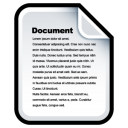
DC Field | Value | Language |
---|---|---|
dc.contributor.advisor | Neitzke, Michael | - |
dc.contributor.author | Vladimirova, Anastasiya | - |
dc.date.accessioned | 2024-01-03T10:46:17Z | - |
dc.date.available | 2024-01-03T10:46:17Z | - |
dc.date.created | 2021-07-22 | - |
dc.date.issued | 2024-01-03 | - |
dc.identifier.uri | http://hdl.handle.net/20.500.12738/14526 | - |
dc.description.abstract | Diese Studie bietet einen umfassenden Überblick über verfügbare Clustering-Methoden und analysiert deren Leistung auf den Merkmalsvektoren von LIDAR-Ceilometerdaten. Nach der Identifizierung relevanter Merkmale der Cluster und der Auswahl der entsprechenden Metriken fährt die Arbeit mit dem Vergleich verfügbarer Methoden zur Partitionierung von Daten fort. In Anbetracht der Tatsache, dass die Daten hochdimensional sind, werden auch die dafür spezialisierten Clustering-Methoden in die Untersuchung einbezogen. Die Studie beinhaltet weiterhin eine Faktorenanalyse der Daten und eine anschließende Reduktion dieser Daten auf niedrigere Dimensionen, die dann demselben Clustering-Analyseprozess unterzogen werden. | de |
dc.description.abstract | This study provides a comprehensive overview of available clustering methods and analyses their performance on the feature vectors of LIDAR-ceilometer data. After identifying relevant characteristics of the clusters and choosing the corresponding metrics, the work proceeds with comparing available methods for partitioning data. Considering that the data is highdimensional, the specialized clustering methods are also included into examination. The study further incorporates factor analysis of the data and subsequent reducing these data to lower dimensions, which then undergo same clustering analysis process. | en |
dc.language.iso | en | en_US |
dc.subject | Clustering | en_US |
dc.subject | Rückstreudaten | en_US |
dc.subject | Dimensionsreduktion | en_US |
dc.subject | Hard-Clustering | en_US |
dc.subject | Spektrales Clustering | en_US |
dc.subject | Hierarchisches Clustering | en_US |
dc.subject | Dichte-basiertes Clustering | en_US |
dc.subject | PCA | en_US |
dc.subject | UMAP | en_US |
dc.subject | T-SNE | en_US |
dc.subject | LIDAR-Ceilometer | en_US |
dc.subject | Unüberwachtes Lernen | en_US |
dc.subject | Hochdimensionales Clustering | en_US |
dc.subject | Subspace-Clustering | en_US |
dc.subject | backscatter data | en_US |
dc.subject | dimensionality reduction | en_US |
dc.subject | spectral clustering | en_US |
dc.subject | hierarchical clustering | en_US |
dc.subject | density-based clustering | en_US |
dc.subject | unsupervised learning | en_US |
dc.subject | subspace clustering | en_US |
dc.subject.ddc | 004: Informatik | en_US |
dc.title | Analysis and Comparison of Various Clustering and Dimensionality Reduction Algorithms on LIDAR-Ceilometer Aerosol-Backscatter Data | en |
dc.type | Thesis | en_US |
openaire.rights | info:eu-repo/semantics/openAccess | en_US |
thesis.grantor.department | Department Informatik | en_US |
thesis.grantor.universityOrInstitution | Hochschule für Angewandte Wissenschaften Hamburg | en_US |
tuhh.contributor.referee | Stelldinger, Peer | - |
tuhh.identifier.urn | urn:nbn:de:gbv:18302-reposit-167555 | - |
tuhh.oai.show | true | en_US |
tuhh.publication.institute | Department Informatik | en_US |
tuhh.publication.institute | Fakultät Technik und Informatik | en_US |
tuhh.type.opus | Bachelor Thesis | - |
dc.type.casrai | Supervised Student Publication | - |
dc.type.dini | bachelorThesis | - |
dc.type.driver | bachelorThesis | - |
dc.type.status | info:eu-repo/semantics/publishedVersion | en_US |
dc.type.thesis | bachelorThesis | en_US |
dcterms.DCMIType | Text | - |
tuhh.dnb.status | domain | en_US |
item.openairecristype | http://purl.org/coar/resource_type/c_46ec | - |
item.fulltext | With Fulltext | - |
item.grantfulltext | open | - |
item.openairetype | Thesis | - |
item.creatorGND | Vladimirova, Anastasiya | - |
item.languageiso639-1 | en | - |
item.creatorOrcid | Vladimirova, Anastasiya | - |
item.cerifentitytype | Publications | - |
item.advisorGND | Neitzke, Michael | - |
Appears in Collections: | Theses |
Files in This Item:
File | Description | Size | Format | |
---|---|---|---|---|
Vladimirova_geschwärzt.pdf | 3.13 MB | Adobe PDF | View/Open |
Note about this record
Export
Items in REPOSIT are protected by copyright, with all rights reserved, unless otherwise indicated.