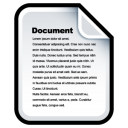
Title: | Analysis and Evaluation of Reinforcement Learning Algorithms for a Continuous Control Problem | Language: | English | Authors: | Babic, Michael | Keywords: | Kontinuierliche Kontrolle; Agenten; Lernende Agenten; Verstärktes Lernen; Machinelles Lernen; Soft Actor Critic; Truncated Quantile Critics; Optuna; OpenAI | Issue Date: | 7-Feb-2024 | Abstract: | Die große Vielfalt an an Reinforcement Learning Algorithmen macht es schwer zu bestimmen, welcher Algorithmus für welche Aufgabe verwendet werden soll. Die wissenschaftlichen Arbeiten, die solche Algorithmen präsentieren, enthalten oft wiedersprüchliche Ergebnisse und machen es dadurch noch schwerer zu verstehen, ob die Erweiterungen der grundlegenden Algorithmen eine Leistungsverbesserung aufweisen. In dieser Arbeit wird ein Ansatz vorgestellt, um eine kontinuierliche Kontrollaufgabe für einen Agenten zu analysieren, eine Gruppe von Algorithmen auf der Grundlage der Merkmale auszuwählen und Sie strukturiert für das Problem zu konfigurieren. Die Konzepte der ausgewählten Algorithmen werden vorgestellt und es wird gezeigt, wie Sie die grundlegenden Reinforcement Learning Algorithmen verbessern, gefolgt von einer Vorhersage Ihrer Leistung in der erwähnten kontinuierlichen Kontrollaufgabe. Weitergehend präsentiert diese Arbeit einen Ansatz zum Finden von Parametern und einer Analyse der Algorithmen, wie schnell funktionierende Parameter gefunden werden konnten und inwiefern welche Veränderungen die Leistung am stärksten beeinflusst hat. Die Abweichung der einzelnen Durchläufen wird durch eine Reevaluierung der besten Parameter Konfigurationen für die Algorithmen reduziert und die stabilsten ausgewählt. Abschließend wird ein detaillierter Einblick in das Verhalten während des Trainings und der Evaluation mit den gewählten Parametern präsentiert, wie die Algorithmen lernen und die Umgebung erkunden, um die Vorhersagen über die Leistung der Algorithmen zu beantworten. Due to the broad variety of Reinforcement Learning algorithms, it is difficult to determine which one to use for what task. Papers that present said algorithms often claim contradictory results which worsens this problem and makes it harder to understand if their extensions of the base algorithms bring an overall improvement in performance. This work presents an approach to analyze a custom created continuous control task, pick an algorithm or a group of algorithms based on found characteristics and provide a structure to configure the algorithms parameters. The concepts of chosen algorithms are shown and how they claim to improve on the basic Reinforcement Learning algorithms, followed by predictions about their performance on the environment. A structured way for finding suitable parameters for the algorithms is presented, and the algorithms are further analyzed, how fast a good set of parameters was found and what changes influenced the performance the most. The run to run variance is reduced by reevaluating the best parameter sets found for each algorithm multiple times and picking the most stable ones. Finally, an in depth view of the algorithms behavior while training and evaluating with found parameter sets is presented, how the algorithms learn and explore, to answer the predictions made about their performance. |
URI: | http://hdl.handle.net/20.500.12738/14777 | Institute: | Department Informatik Fakultät Technik und Informatik |
Type: | Thesis | Thesis type: | Bachelor Thesis | Advisor: | Neitzke, Michael | Referee: | Becke, Martin |
Appears in Collections: | Theses |
Files in This Item:
File | Description | Size | Format | |
---|---|---|---|---|
Analysis and Evaluation of Reinforcement Learning_geschwärzt.pdf | 2.32 MB | Adobe PDF | View/Open |
Note about this record
Export
Items in REPOSIT are protected by copyright, with all rights reserved, unless otherwise indicated.