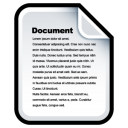
Title: | Detecting Uncertainty in Text Classifications : A Sequence to Sequence Approach using Bayesian RNNs | Language: | English | Authors: | Schöner, Tom | Keywords: | Unsicherheit; Textklassifikation; NLP; Sequenzanalyse; Bayesian LSTM | Issue Date: | 17-May-2024 | Abstract: | Deep Learning Modellen liegen Unsicherheiten zu Grunde, die häufig unbeachtet bleiben oder fehlinterpretiert werden. Die Zusammenführung von Recurrent Neural Networks (RNNs) und Bayesschen Methoden ist eine Möglichkeit, diese Unsicherheiten zu ermitteln. Am Beispiel der Textklassifikation evaluiert diese Arbeit einen neuartigen Ansatz, um Unsicherheitswerte auf Basis von Tokens zu quantifizieren. Ziel ist es, eine transparente Auswertung der Eingabesequenzen zu ermöglichen. Uncertainty is fundamentally connected to deep learning but is often disregarded or misinterpreted. Combining Recurrent Neural Networks (RNNs) with Bayesian methods is one way to detect these uncertainties. This work evaluates a novel approach to quantify uncertainty on a token-basis for text classification. The goal is to enable a transparent evaluation of input sequences. |
URI: | http://hdl.handle.net/20.500.12738/15757 | Institute: | Fakultät Technik und Informatik Department Informatik |
Type: | Thesis | Thesis type: | Master Thesis | Advisor: | Zukunft, Olaf | Referee: | Tropmann-Frick, Marina ![]() |
Appears in Collections: | Theses |
Files in This Item:
File | Description | Size | Format | |
---|---|---|---|---|
MA_Detecting_Uncertainty.pdf | 2.5 MB | Adobe PDF | View/Open |
Note about this record
Export
Items in REPOSIT are protected by copyright, with all rights reserved, unless otherwise indicated.