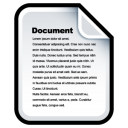
Title: | Comparison of Data Augmentation Techniques for efficient Training of Image-Text Classification Algorithms | Language: | English | Authors: | Ostrovskiy, Nikita | Keywords: | Maschinelles Lernen; CNN; Neuronales Netzwerk; Datenerweiterung; Bildverarbeitung; Bild- Text-Klassifikation; Machine Learning; Neural Network; Data augmentation; Image Processing; Image-Text Classification | Issue Date: | 5-Jun-2024 | Abstract: | In diesem Beitrag werden verschiedene Methoden zur Datenerweiterung vorgestellt, die beim Training eines CNN zur Bild-Text-Klassifikation eingesetzt werden. Die Prinzipien, Konzepte, Anwendungen sowie die Effektivität dieser Methoden werden auf der Grundlage bereits durchgeführter wissenschaftlicher Arbeiten diskutiert. Dabei werden sowohl gängige Methoden als auch komplexere und noch weniger erforschte Methoden behandelt. Im experimentellen Teil dieser Arbeit wird ein CNN erstellt, das den GTSRB-Datensatz zum Erlernen der Bild-Text- Klassifikation verwendet. Einige der in dieser Arbeit besprochenen Methoden werden angewendet, um den Datensatz zu erweitern, so dass wir ihre Auswirkungen auf das Training und das Ausmaß, in dem sie bei der Aufgabe, für die sie entwickelt wurden, helfen, sehen können. This paper introduces the reader to the different data augmentation methods used in training of a convolutional neural networks that conducts image-text classifcations. The principles, concepts, applications, as well as the effectiveness of these methods will be discussed on the basis of already conducted scientific work. Commonly used methods as well as more complex and even less researched methods will be covered in this work. In the experimental part of this paper, a convolutional neural network will be created that will use the GTSRB dataset to learn image-text classification. Some of the methods discussed in this paper will be applied in order to augment the dataset, allowing us to see their effect on training and the extent to which they help with the task for which they were developed. |
URI: | http://hdl.handle.net/20.500.12738/15878 | Institute: | Department Informatik Fakultät Technik und Informatik |
Type: | Thesis | Thesis type: | Bachelor Thesis | Advisor: | Tropmann-Frick, Marina ![]() |
Referee: | Schultz, Martin ![]() |
Appears in Collections: | Theses |
Files in This Item:
File | Description | Size | Format | |
---|---|---|---|---|
BA_Comparison of Data Augmentation Techniques for efficient Training of Image-Text Classification Algorithms_geschwärzt.pdf | 7.57 MB | Adobe PDF | View/Open |
Note about this record
Export
Items in REPOSIT are protected by copyright, with all rights reserved, unless otherwise indicated.