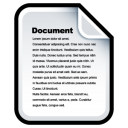
Title: | Global Contextualized Representations: Enhancing Machine Reading Comprehension with Graph Neural Networks | Language: | English | Authors: | Tran, Thien Phuc | Keywords: | Natural Language Processing; Transformers; Graph Neural Networks; Knowledge Graphs; Question Answering; Machine Reading Comprehension | Issue Date: | 2-Aug-2024 | Abstract: | In dieser Arbeit werden globale kontextualisierte Repräsentationen (GCoRe) vorgestellt - ein Zusatzmodul zu bestehenden Transformer-basierten Sprachmodellen, das die Leistung bei Question-Answering / Machine-Reading - Comprehension Aufgaben verbessert. Während Transformer-basierte Modelle eine begrenzte Eingabelänge haben und oft nicht in der Lage sind, globalen Kontext und weitreichende Abhängigkeiten zu erfassen, wird GCoRe entwickelt, um diese Probleme zu entschärfen, indem Graph-Inferenz mit Graph Neural Networks auf einem Kontextgraphen durchgeführt wird, der aus dem gesamten Eingabetext konstruiert wurde. Die kontextualisierten Token-Embeddings werden dann mit den aus dem Kontextgraphen abgeleiteten globalen kontextualisierten Merkmalen angereichert. Die Experiment-Ergebnisse zeigen, dass GCoRe das Basismodell im HotpotQA-Datensatz, der aus komplexen Fragen und langen Kontexttexten besteht, um 0,57% übertrifft. Auch im SQuAD 2.0-Datensatz, der kürzere Absätze und Single-Hop-Fragen enthält, übertrifft GCoRe das Basismodell um 0.15%. Besonders bemerkenswert ist, dass GCoRe eine Frage, die Schlussfolgerungen über familiäre Beziehungen erfordert, korrekt beantwortet, während das Basismodell DeBERTa v3 die Frage nicht korrekt beantworten kann. This thesis introduces Global Contextualized Representations (GCoRe) – an add-on module to existing Transformer-based language models, which helps improve the performance on Question Answering / Machine Reading Comprehension tasks. Whereas Transformerbased models have limited input length and often fail to capture global context and long-range dependencies, GCoRe is designed to mitigate these problems by performing graph inference using Graph Neural Networks on a context graph constructed from the entire input text. The contextualized token embeddings are then augmented with the inferred global contextualized features derived from the context graph. The experimental results demonstrate that GCoRe surpasses the baseline model by 0.57% in the HotpotQA dataset, which comprises complex questions and lengthy context texts. GCoRe also outperforms the baseline model by 0.15% in the SQuAD 2.0 dataset which contains shorter paragraphs and single-hop questions. Notably, GCoRe accurately answers a question that requires reasoning about familial relationships, while the baseline model, DeBERTa v3, fails to answer the question correctly. |
URI: | https://hdl.handle.net/20.500.12738/16131 | Institute: | Fakultät Technik und Informatik Department Informatik |
Type: | Thesis | Thesis type: | Master Thesis | Advisor: | Tropmann-Frick, Marina ![]() |
Referee: | Sarstedt, Stefan |
Appears in Collections: | Theses |
Files in This Item:
File | Description | Size | Format | |
---|---|---|---|---|
MA_Global Contextualized Representations.pdf | 2.25 MB | Adobe PDF | View/Open |
Note about this record
Export
Items in REPOSIT are protected by copyright, with all rights reserved, unless otherwise indicated.