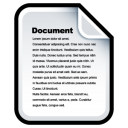
Titel: | Prediction of depressive episodes using biomedical sensors with machine learning techniques | Sprache: | Englisch | Autorenschaft: | Hussen, Hesham | Schlagwörter: | Machine learning; Depression Detection; Data preprocessing; Health Data; CNN; RNN; GRU; Random Forest; Ensemble Methods; Linear SVR; Python; Tensorflow; Maschinelles Lernen; Depression Vorhersagen; Data Vorverarbeitung; Ensemblemethoden | Erscheinungsdatum: | 13-Sep-2024 | Zusammenfassung: | The behaviours of patients with depression are usually difficult to predict because the patients demonstrate the symptoms of a depressive episode without warning at unexpected times. The goal of this thesis is to examine different machine learning approaches to detect such times and predict possible depressive episodes. The work also comprises a preprocessing pipeline for transforming and organizing the input data The final assessment indicates current and future application possibilities of machine learning in the depression detection context. Das Verhalten von Patienten mit einer Depression ist in der Regel schwer vorherzusagen, da die Patienten die Symptome einer depressiven Episode ohne Vorwarnung zu unerwarteten Zeiten zeigen. Das Ziel dieser Arbeit ist es, verschiedene Ansätze des maschinellen Lernens zu untersuchen, um solche Zeiten zu erkennen und mögliche depressive Episoden vorherzusagen. Die Arbeit umfasst auch eine Vorverarbeitungs-Pipeline zur Transformation und Organisation der Eingabedaten. Die abschliessende Bewertung zeigt aktuelle und zukünftige Anwendungsmöglichkeiten des maschinellen Lernens im Kontext der Depressionserkennung auf. |
URI: | https://hdl.handle.net/20.500.12738/16256 | Einrichtung: | Fakultät Technik und Informatik Department Informations- und Elektrotechnik |
Dokumenttyp: | Abschlussarbeit | Abschlussarbeitentyp: | Bachelorarbeit | Hauptgutachter*in: | Dahlkemper, Jörg | Gutachter*in der Arbeit: | Martens, Andreas |
Enthalten in den Sammlungen: | Theses |
Dateien zu dieser Ressource:
Datei | Beschreibung | Größe | Format | |
---|---|---|---|---|
BA_Prediction of depressive episodes_medical sensors_machine learning.pdf | 2.57 MB | Adobe PDF | Öffnen/Anzeigen |
Feedback zu diesem Datensatz
Export
Alle Ressourcen in diesem Repository sind urheberrechtlich geschützt.