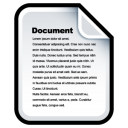
Title: | Combining image inpainting and optical flow for integration of pathologies to deep learning-based endoscopic trainings simulators | Language: | English | Authors: | Wallrodt, Moritz Tizian | Keywords: | Deep Learning; Endoskopische Trainingsimulatoren; Generative Adversarial Networks; Diffusion Models; Bildkomposition | Issue Date: | 13-Sep-2024 | Abstract: | Endoskopische Simulatoren für das Training von Untersuchungen des Magen-Darm-Trakts werden üblicherweise aus Silikon hergestellt. Trotz einer qualitativ guten Nachbildung der Organe, bleibt das äußere Erscheinungsbild und das Trainingsgefühl weit von einer echten Untersuchung entfernt, wobei das Szenario bei jeder Untersuchung identisch bleibt. Aktuelle DeepLearning-Ansätze können Bilder von Trainingssimulatoren in synthetische Ansichten umwandeln, bisher jedoch meist nur für die Darstellung unauffälliger gesunder Schleimhaut. In dieser Arbeit wird das Potenzial von Generative Adversarial Nets (GANs) und Denoising Diffusion Probabilistic Models (DDPMs) zur Integration von Pathologien in synthetische Ansichten gesunder Schleimhaut und deren zeitkonsistente Verschiebung in kurzen Videosequenzen mittels Optical Flow untersucht. DDPMs liefern qualitativ hochwertige Ergebnisse beim Einzeichnen von Pathologien, haben jedoch eine signifikant längere Inferenzzeit als GAN-basierte Modelle. Der Optical Flow ermöglicht das Erstellen kurzer, konsistenter Videosequenzen, zeigt jedoch Limitationen bei der Anpassung der Lichtverhältnisse und Positionierung von Pathologien. Die Ergebnisse legen nahe, für zukünftige Methodenentwicklungen Pathologien auf Einzelbildebene einzuzeichnen und nur die dafür verwendeten Masken mit Optical Flow zu transformieren, statt - wie in dieser Arbeit - die Pathologie selbst. Ferner sollte die Implementierung der Modelle in Trainingssimulatoren weiter erforscht werden. Endoscopic simulators for training examinations of the gastrointestinal tract are usually made of silicone. Despite a good quality replica of the organs, the external appearance and training experience remains far away from a real examination, with the scenario remaining identical for each examination. Current deep learning approaches can convert images of training simulators into synthetic views, but so far mostly for the visualization of inconspicuous healthy mucosa. This work investigates the potential of Generative Adversarial Nets (GANs) and Denoising Diffusion Probabilistic Models (DDPMs) to integrate pathologies into synthetic views of healthy mucosa and their time-consistent displacement in short video sequences using optical flow. DDPMs provide high quality results when drawing pathologies, but have a longer inference time than GAN-based models. Optical flow enables the creation of short, consistent video sequences, but shows limitations in the adjustment of lighting conditions and positioning of pathologies. The results suggest that for future method developments, pathologies should be drawn at the single image level and only the masks used for this should be transformed with Optical Flow instead of the pathology itself, as done in this work. Furthermore, the integration of the models to training simulators should be further researched. |
URI: | https://hdl.handle.net/20.500.12738/16262 | Institute: | Fakultät Life Sciences Department Medizintechnik |
Type: | Thesis | Thesis type: | Master Thesis | Advisor: | Schiemann, Thomas | Referee: | Schmitz, Rüdiger |
Appears in Collections: | Theses |
Files in This Item:
File | Description | Size | Format | |
---|---|---|---|---|
MA_Combining_image_inpainting_optical_flow.pdf | 2.32 MB | Adobe PDF | View/Open |
Note about this record
Export
Items in REPOSIT are protected by copyright, with all rights reserved, unless otherwise indicated.