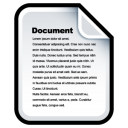
DC Element | Wert | Sprache |
---|---|---|
dc.contributor.advisor | Taefi, Tessa | - |
dc.contributor.author | Hesse, Mira | - |
dc.date.accessioned | 2024-09-20T09:24:31Z | - |
dc.date.available | 2024-09-20T09:24:31Z | - |
dc.date.created | 2024-05-23 | - |
dc.date.issued | 2024-09-20 | - |
dc.identifier.uri | https://hdl.handle.net/20.500.12738/16303 | - |
dc.description.abstract | There are several approaches to bat call classification using deep learning. In this thesis, I compared existing architectures for bat call classification and for general audio classification on a dataset that contained acoustic drone noise in the recordings in addition to bat calls. Two pretrained models (without further training) showed no significant difference between data with and without drone noise. I also compared two pretrained models after transfer learning. For the training dataset I compared different levels of resampling and an augmented dataset to compensate for the high class imbalance between the three classes in the dataset. The best results were obtained using the Patchout faSt Spectrogram Transformer (PaSST) model with oversampling. The model achieved a f1-score of 94.9% on a binary classification task. On multilabel classification it achieved a micro f1-Score of 90.6% and a macro f1-score of 78.5%. | en |
dc.description.abstract | Es gibt bereits einige Modelle für die Klassifikation von Fledermausrufen mithilfe von Deep Learning. In dieser Arbeit habe ich existierende Modelle für die Klassifizierung von Fledermausrufen und für die allgemeine Audioklassifikation für einen Datensatz verglichen, der zusätzlich akustisches Drohnenrauschen enthält. Zwei vortrainierte Modelle (ohne weiteres Training) zeigten keinen signifikanten Unterschied zwischen Daten mit und ohne Drohnenrauschen. Ich habe auch zwei Modelle nach „Transfer Learning“ verglichen. Für den Trainingsdatensatz habe ich verschiedene „Resampling“ und „Augmentation“ Kombinationen verglichen, um das hohe Ungleichgewicht zwischen den drei Klassen im Datensatz auszugleichen. Die besten Ergebnisse wurden mit dem Modell „Patchout faSt Spectrogram Transformer“ (PaSST) und „Oversampling“ erzielt. Das Modell erreichte einen F1-Score von 94, 3% für binäre Klassifizierung. Für „Multilabel“ Klassifizierung erreichte es einen Mikro F1-Score von 90, 6% und einen Makro F1-Score von 78, 5%. | de |
dc.language.iso | en | en_US |
dc.subject.ddc | 004: Informatik | en_US |
dc.title | Bat Call Classification in Audio Recordings with Drone Noise Using Deep Learning | en |
dc.type | Thesis | en_US |
openaire.rights | info:eu-repo/semantics/openAccess | en_US |
thesis.grantor.department | Fakultät Design, Medien und Information | en_US |
thesis.grantor.department | Department Medientechnik | en_US |
thesis.grantor.department | Competence Center Erneuerbare Energien und Energieeffizienz | en_US |
thesis.grantor.universityOrInstitution | Hochschule für Angewandte Wissenschaften Hamburg | en_US |
tuhh.contributor.referee | Roswag, Marc | - |
tuhh.identifier.urn | urn:nbn:de:gbv:18302-reposit-194941 | - |
tuhh.oai.show | true | en_US |
tuhh.publication.institute | Fakultät Design, Medien und Information | en_US |
tuhh.publication.institute | Department Medientechnik | en_US |
tuhh.publication.institute | Competence Center Erneuerbare Energien und Energieeffizienz | en_US |
tuhh.type.opus | Bachelor Thesis | - |
dc.type.casrai | Supervised Student Publication | - |
dc.type.dini | bachelorThesis | - |
dc.type.driver | bachelorThesis | - |
dc.type.status | info:eu-repo/semantics/publishedVersion | en_US |
dc.type.thesis | bachelorThesis | en_US |
dcterms.DCMIType | Text | - |
tuhh.dnb.status | domain | en_US |
item.creatorGND | Hesse, Mira | - |
item.grantfulltext | open | - |
item.openairetype | Thesis | - |
item.advisorGND | Taefi, Tessa | - |
item.fulltext | With Fulltext | - |
item.languageiso639-1 | en | - |
item.cerifentitytype | Publications | - |
item.creatorOrcid | Hesse, Mira | - |
item.openairecristype | http://purl.org/coar/resource_type/c_46ec | - |
Enthalten in den Sammlungen: | Theses |
Dateien zu dieser Ressource:
Datei | Beschreibung | Größe | Format | |
---|---|---|---|---|
BA_Bat_Call_Classification_in_Audio_Recordings.pdf | 1.03 MB | Adobe PDF | Öffnen/Anzeigen |
Feedback zu diesem Datensatz
Export
Alle Ressourcen in diesem Repository sind urheberrechtlich geschützt.