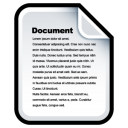
DC Field | Value | Language |
---|---|---|
dc.contributor.advisor | von Luck, Kai | - |
dc.contributor.author | Ottenheym, Thore | - |
dc.date.accessioned | 2025-01-02T12:07:51Z | - |
dc.date.available | 2025-01-02T12:07:51Z | - |
dc.date.created | 2024-09-13 | - |
dc.date.issued | 2025-01-02 | - |
dc.identifier.uri | https://hdl.handle.net/20.500.12738/16770 | - |
dc.description.abstract | Diese thesis untersucht die Anwendbarkeit von Graph Convolutional Networks (GCNs) zur Automatisierung der Interpretation von in-the-wild Skelettdaten. Die Studie untersucht, ob GCNs, insbesondere das Spatial-Temporal Graph Convolutional Network (ST-GCN), Gesten, die in einer unkontrollierten Umgebung aufgenommen wurden, effizient erkennen können. Es wurde ein Datensatz mit Daten aus dem HopE-Projekt erstellt und eine Trainingspipeline entwickelt, die Techniken des Transfer learnings und der Data Augmentation beinhaltet. Die Ergebnisse zeigen, dass GCNs in der Lage sind, die räumliche und zeitliche Dynamik zu erfassen, die für eine genaue Gestenerkennung in realen Szenarien notwendig ist, und geben einen Einblick in das Potenzial von GCNs zur Verbesserung der automatischen Gesteninterpretation in unterschiedlichen und unvorhersehbaren Umgebungen. | de |
dc.description.abstract | This thesis investigates the applicability of Graph Convolutional Networks (GCNs) for automating the interpretation of in-the-wild skeletal data. The study examines whether GCNs, specifically the Spatial-Temporal Graph Convolutional Network (ST-GCN), can effectively interpret gestures captured in uncontrolled environments. A dataset was created using data from the HopE project, and a training pipeline was developed that incorporates transfer learning and data augmentation techniques. The results demonstrate that GCNs can capture the spatial and temporal dynamics necessary for accurate gesture recognition in real-world scenarios, and provide insight into the potential of GCNs to improve automated gesture interpretation in diverse and unpredictable environments. | en |
dc.language.iso | en | en_US |
dc.subject | Skelettdaten | en_US |
dc.subject | Graph Convolution Network | en_US |
dc.subject | Gesture Interpretation | en_US |
dc.subject | Skeleton data | en_US |
dc.subject.ddc | 004: Informatik | en_US |
dc.title | Graph Convolutional Network based Gesture Interpretation of Sekeleton Data | en |
dc.type | Thesis | en_US |
openaire.rights | info:eu-repo/semantics/openAccess | en_US |
thesis.grantor.department | Fakultät Technik und Informatik | en_US |
thesis.grantor.department | Department Informatik | en_US |
thesis.grantor.universityOrInstitution | Hochschule für Angewandte Wissenschaften Hamburg | en_US |
tuhh.contributor.referee | Schwarzer, Jan | - |
tuhh.identifier.urn | urn:nbn:de:gbv:18302-reposit-201865 | - |
tuhh.oai.show | true | en_US |
tuhh.publication.institute | Fakultät Technik und Informatik | en_US |
tuhh.publication.institute | Department Informatik | en_US |
tuhh.type.opus | Bachelor Thesis | - |
dc.type.casrai | Supervised Student Publication | - |
dc.type.dini | bachelorThesis | - |
dc.type.driver | bachelorThesis | - |
dc.type.status | info:eu-repo/semantics/publishedVersion | en_US |
dc.type.thesis | bachelorThesis | en_US |
dcterms.DCMIType | Text | - |
tuhh.dnb.status | domain | en_US |
item.advisorGND | von Luck, Kai | - |
item.grantfulltext | open | - |
item.languageiso639-1 | en | - |
item.openairetype | Thesis | - |
item.openairecristype | http://purl.org/coar/resource_type/c_46ec | - |
item.fulltext | With Fulltext | - |
item.creatorGND | Ottenheym, Thore | - |
item.creatorOrcid | Ottenheym, Thore | - |
item.cerifentitytype | Publications | - |
Appears in Collections: | Theses |
Files in This Item:
File | Description | Size | Format | |
---|---|---|---|---|
BA_Graph Convolutional Network.pdf | 8.64 MB | Adobe PDF | View/Open |
Note about this record
Export
Items in REPOSIT are protected by copyright, with all rights reserved, unless otherwise indicated.