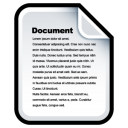
Title: | Detektion von Sprachbefehlen auf Edge-Geräten unterstützt durch automatisierte Trainingsdaten-Synthese für eine Not-Halt-Anwendung | Language: | German | Authors: | Sentler, Dennis | Keywords: | Edge Computing; Künstliche Intelligenz; AI on Edge; Automatisiertes Training; Robotik; Smart City; Not-Halt; Sprachsteuerung; Mensch-Maschinen-Interaktion; Artificial Intelligence; Automated Training; Robotics; Emergency Stop; Voice Control; Human–Machine Interface | Issue Date: | 12-Mar-2025 | Abstract: | Ziel dieser Arbeit ist es, einen durch Sprachbefehle gesteuerten Not-Halt-Kontroller zu entwickeln, der auf einem Edge-Gerät mit geringem Leistungsvermögen betrieben werden kann. Sekundäres Ziel ist eine Trainingsanwendung, die den aufwendigen Trainingsprozess mithilfe von synthetischen Trainingsdaten automatisieren kann. Dabei stellen sich zusätzliche Fragen: Welche neuronale Netzwerkarchitektur eignet sich für eine Herunterskalierung auf Edge-Geräte-Niveau? Kann State of the Art Sprachsynthese den manuellen Arbeitsaufwand im vorliegenden Fall ersetzen? Es ist möglich, dass für neuronale Netze ein synthetischer Datensatz noch kein voller Ersatz ist. Andererseits hat die Sprachsynthese in den letzten Jahren große Fortschritte gezeigt. Für die Umsetzung wird eine aus dem MobileNet [1] bekannte DS-CNN-Architektur verwendet und herunterskaliert. Das Training wird auf dem Google Speech Command Dataset [2] sowie auf einem synthetischen Datensatz umgesetzt. Die Audiosynthese erfolgt über eine eigene GUI-Anwendung, die mit führenden Online-TTS-Anbietern integriert ist. Es stellt sich heraus, dass die DS-CNN-Architektur grundsätzlich für diesen Kontext geeignet ist, die Trainingsdaten aber über den Erfolg bestimmen. Im Vergleich können die allein synthetisch-trainierten Netzwerke bei der Detektion nicht mithalten. Allerdings konnte mit dem Google Speech Command Dataset [2] der Not-Halt-Kontroller erfolgreich umgesetzt werden. The goal of this work is to develop an emergency stop controller operated by voice commands that can be deployed on an edge device with a small footprint. The secondary goal is a training application that automates the time-consuming training process using synthetic training data. Several questions arise: Which neural network architecture is suitable for downscaling to edge device capabilities? Can state-of-the-art speech synthesis replace the need to manually collect and categorize speech recordings in our case? It is possible that for neural networks, a synthetic dataset is still not a full substitute. On the other hand, speech synthesis has shown growing potential in recent years. For the implementation, a downscaled DS-CNN architecture known from MobileNet [1] is used. Training is performed on the Google Speech Command Dataset [2] and on a synthetic dataset. Audio synthesis is accomplished using a custom GUI application integrated with leading online TTS providers. It is found that the DS-CNN architecture is fundamentally suitable for this context, but the training data dictates its success; the networks trained on only synthetic data cannot keep up with those trained on real audio data. However, by using the Google Speech Command Dataset [2], the emergency stop controller has been successfully implemented. |
URI: | https://hdl.handle.net/20.500.12738/17294 | Institute: | Department Informatik Fakultät Technik und Informatik |
Type: | Thesis | Thesis type: | Bachelor Thesis | Advisor: | Pareigis, Stephan ![]() |
Referee: | Stelldinger, Peer ![]() |
Appears in Collections: | Theses |
Files in This Item:
File | Description | Size | Format | |
---|---|---|---|---|
BA_Detektion von Sprachbefehlen auf Edge-Geräten_geschwärzt.pdf | 2.27 MB | Adobe PDF | View/Open |
Note about this record
Export
Items in REPOSIT are protected by copyright, with all rights reserved, unless otherwise indicated.