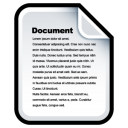
Title: | Raum-Koordinatenbestimmung durch Deep-Learning-Analyse von rasterbasierten Grundrissplänen | Language: | German | Authors: | Akhtar, Zafar | Keywords: | Instance-Segmentierung; Deep-Learning; rasterbasierte Grundrisspläne; Harris-Corner- Detector; Instance segmentation; Deep learning; Bitmap floor plan | Issue Date: | 3-Apr-2025 | Abstract: | Ein Grundrissplan ist ein Bestandteil von Bauzeichnungen und stellt eine maßstabsgetreue zeidimensionale Abbildung der Räumlichkeiten einer Etage da. In dieser Arbeit wurde die Anwendbarkeit von Deep-Learning mit Schwerpunkt Instanz-Segmentierung auf rasterbasierte Grundrisspläne untersucht, um so Eckpunkte eines Raums zu bestimmen. Es wurde ein mehrstufiges System konzipiert, bei welchem das Mask R-CNN Modell Masken von Räumen erzeugt, welche anschließend mit dem Harris-Corner-Detector nach Ecken abgesucht werden. Grundlage der Daten bilden dabei frei zugängliche als auch ein selbst annotierter Datensatz. In den Experimenten wurde das Mask R-CNN quantitativ evaluiert und Vergleiche zwischen unterschiedlichen Konfigurationen vorgenommen. Anschließend wurden Ergebnisse qualitativ ausgewertet. Es stellte sich heraus, dass das System überwiegend Räume als solches detektieren und segmentieren kann. Jedoch sind Grundrisspläne mit vielen Störquellen ein Hindernis, weshalb Masken in ungleichmäßigen Dimensionen erzeugt werden. Werden Masken gleichmäßig erzeugt, können Ecken effizienter lokalisiert werden. A floor plan is part of architectural drawings and represents a true-to-scale, two-dimensional representation of the premises on a floor. In this work, the applicability of deep learning with a focus on instance segmentation on bitmap floor plans was examined in order to determine corner points of a room. A multi-stage system was designed in which the Mask R-CNN model generates masks of rooms and these are then searched for corners with the Harris-Corner-Detector. The data is based on freely accessible and self-annotated data sets. In the experiments, the Mask R-CNN was evaluated quantitatively and comparisons were made between different configurations. The results were then evaluated qualitatively. It turned out that the system can predominantly detect and segment rooms as such. However, floor plans with many sources of interference are an obstacle, which is why masks are generated in non-uniform dimensions. If masks are generated evenly, corners can be located more efficiently. |
URI: | https://hdl.handle.net/20.500.12738/17354 | Institute: | Fakultät Technik und Informatik Department Informatik |
Type: | Thesis | Thesis type: | Bachelor Thesis | Advisor: | Pareigis, Stephan ![]() |
Referee: | Neitzke, Michael |
Appears in Collections: | Theses |
Files in This Item:
File | Description | Size | Format | |
---|---|---|---|---|
BA_Raum-Koordinatenbestimmung durch Deep-Learning-Analyse.pdf | 8.35 MB | Adobe PDF | View/Open |
Note about this record
Export
Items in REPOSIT are protected by copyright, with all rights reserved, unless otherwise indicated.