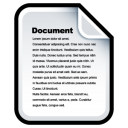
Titel: | Evaluating Saliency Map Methods in Reinforcement Learning | Sprache: | Englisch | Autorenschaft: | Helmboldt, Rico | Schlagwörter: | Erklärbare KI; XAI; Salienzkarten; Pixel Attribution; Deletion | Erscheinungsdatum: | 4-Apr-2025 | Zusammenfassung: | Die Salienzkartenmethoden Vanilla Gradients, SmoothGrad, (guided-) GradCAM, LIME und RisE werden quantitativ in Bezug auf ihre Korrektheit evaluiert. Dies wird in einem verstärkenden Lernen Setting mit DQN und Atari Breakout umgesetzt. Zur quantitativen Evaluierung wird Deletion verwendet, wobei der Performanceverlust des Agenten gemessen wird. Es wird gezeigt, dass LRP die Korrekteste Methode ist, aber zusammen mit RisE verwendet werden sollte, um eine vollständigere Erklärung zu erhalten. The saliency map methods Vanilla Gradients, SmoothGrad, (guided-) GradCAM, LIME and RisE are evaluated quantitatively with regards to their correctness. This is done in a reinforcement learning setting with DQN and Atari Breakout. As means to evaluate them quantitatively, Deletion is applied to measure how quickly the performance dwindles. It is shown that LRP is the most correct saliency map method, but should be used together with RisE for a more complete explanation. |
URI: | https://hdl.handle.net/20.500.12738/17364 | Einrichtung: | Fakultät Technik und Informatik Department Informatik |
Dokumenttyp: | Abschlussarbeit | Abschlussarbeitentyp: | Masterarbeit | Hauptgutachter*in: | Tropmann-Frick, Marina ![]() |
Gutachter*in der Arbeit: | Pareigis, Stephan ![]() |
Enthalten in den Sammlungen: | Theses |
Dateien zu dieser Ressource:
Datei | Beschreibung | Größe | Format | |
---|---|---|---|---|
BA_Evaluating_Saliency_Map_Methods_in_Reinforcement_Learning.pdf | 5.82 MB | Adobe PDF | Öffnen/Anzeigen |
Feedback zu diesem Datensatz
Export
Alle Ressourcen in diesem Repository sind urheberrechtlich geschützt.