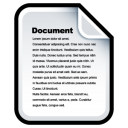
License: | ![]() |
Title: | Adaptiver Neuro-Fuzzy-Ansatz zur Diagnose | Language: | German | Authors: | Yildirim, Feridun | Issue Date: | 30-May-2017 | Abstract: | In der realen Computerumgebung ist es schwierig, eine Entscheidung zu treffen, die durch Unvollständigkeit und Ungenauigkeit charakterisiert ist. Mehrere Algorithmen und Technologien (Fuzzy-Logik, neuronale Netze, genetische Algorithmen etc.) wurden entwickelt, um eine akkurate Diagnose sicher zu stellen. In dieser Bachelor-arbeit wird ein adaptiver Neuro-Fuzzy-Ansatz als Klassifikator für eine Diagnose benutzt. Viele Aspekte des Adaptiven Neuro-Fuzzy-Inferenzsystems (ANFIS) wie Architektur, Variationen der Architektur, Lernalgorithmen, Implementationen und medizinische Anwendungen werden vorgestellt. Für eine Realisierung an einem Fall-beispiel wurden mehrere Modelle mit unterschiedlichen Konfigurationen entwickelt und evaluiert. Die Leistung der Modelle sind mit der Wurzel der mittleren quadrati-schen Fehler (RMSE) ermittelt, um das beste Modell zu erhalten. Die Analyse der Diagnoseresultate und die Vergleiche mit MLP/RBFN demonstrieren eine vielver-sprechende Leistung für eine Modellierung von Diagnosesystemen. It is difficult to derive a decision in a real-world computing environment, which is characterized by incompleteness, inaccuracy and imprecision. Several algorithms and technologies (soft computing techniques - fuzzy logic, neural networks, genetic algo-rithm etc.) have been constructed to ensure accurate diagnosis. In this thesis, an adaptive neuro-fuzzy approach is used as a classifier for diagnosis. Many aspects of the proposed adaptive neuro-fuzzy inference system (ANFIS) are introduced such as architecture, variations, learning algorithms, implementations and medical diag-nostic applications. For case example, several models with different configurations are developed and evaluated. The performance of these models was measured by root-mean-square error (RMSE) to obtain the best fit model. Analysis of the diagnosis results and comparisons with MLP/RBFN demonstrates a promising performance for modeling of diagnostic systems. |
URI: | http://hdl.handle.net/20.500.12738/7980 | Institute: | Department Informatik | Type: | Thesis | Thesis type: | Bachelor Thesis | Advisor: | Meisel, Andreas | Referee: | Fohl, Wolfgang |
Appears in Collections: | Theses |
Files in This Item:
File | Description | Size | Format | |
---|---|---|---|---|
BA_ANFIS_2017_Yildirim_Feridun.pdf | 2.82 MB | Adobe PDF | View/Open |
Note about this record
Export
Items in REPOSIT are protected by copyright, with all rights reserved, unless otherwise indicated.