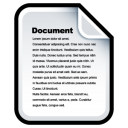
DC Field | Value | Language |
---|---|---|
dc.contributor.advisor | Renz, Wolfgang | - |
dc.contributor.author | Ray, Amrit | |
dc.date.accessioned | 2020-09-29T14:22:24Z | - |
dc.date.available | 2020-09-29T14:22:24Z | - |
dc.date.created | 2017 | |
dc.date.issued | 2017-08-08 | |
dc.identifier.uri | http://hdl.handle.net/20.500.12738/8119 | - |
dc.description.abstract | - | de |
dc.description.abstract | Diverse deep neural network (DNN) approaches have displayed high accuracy in the fields of pattern recognition and image classification but their potential has not been explored in the field of energy disaggregation. The aim of this thesis is to investigate the accuracy with which two DNN approaches classify active household appliances for energy disaggregation and compare the performance of DNN with other classification methods used in the field. The first approach used is the Multi-Layer Perceptron (MLP) approach which is one of the simplest DNN methods and it displays baseline accuracy of any DNN. The second approach is Convolutional Neural Networks (CNN), which is more advanced and improves upon the baseline accuracy. Both approaches are tested with various optimizers, activation functions and loss functions as performance measures. Open source data (REDD dataset) is used to train and test the neural networks. The dataset consists of 6 houses which are used for training and the testing 3 labelled appliances common among the houses (dishwasher, lighting and washer dryer) with the addition of unknown appliance data. When presented with real world data which included unknown devices in addition to the three labelled devices, CNN achieved an accuracy of 90.00% and MLP achieved an accuracy of 77.14%. When the experiment was repeated with data including only the known devices, CNN achieved an accuracy of 95.83% and MLP achieved 80.37%. | en |
dc.language.iso | de | de |
dc.rights.uri | http://rightsstatements.org/vocab/InC/1.0/ | - |
dc.subject | Energy Disaggregation | en |
dc.subject | Deep Neural Networks | en |
dc.subject.ddc | 621.3 Elektrotechnik, Elektronik | |
dc.title | Energy Disaggregation using Deep Neural Networks on Household Appliances | de |
dc.type | Thesis | |
openaire.rights | info:eu-repo/semantics/openAccess | |
thesis.grantor.department | Department Informations- und Elektrotechnik | |
thesis.grantor.place | Hamburg | |
thesis.grantor.universityOrInstitution | Hochschule für angewandte Wissenschaften Hamburg | |
tuhh.contributor.referee | Rohjans, Sebastian | - |
tuhh.gvk.ppn | 895177617 | |
tuhh.identifier.urn | urn:nbn:de:gbv:18302-reposit-81218 | - |
tuhh.note.extern | publ-mit-pod | |
tuhh.note.intern | 1 | |
tuhh.oai.show | true | en_US |
tuhh.opus.id | 4067 | |
tuhh.publication.institute | Department Informations- und Elektrotechnik | |
tuhh.type.opus | Bachelor Thesis | - |
dc.subject.gnd | Energie | |
dc.type.casrai | Supervised Student Publication | - |
dc.type.dini | bachelorThesis | - |
dc.type.driver | bachelorThesis | - |
dc.type.status | info:eu-repo/semantics/publishedVersion | |
dc.type.thesis | bachelorThesis | |
dcterms.DCMIType | Text | - |
tuhh.dnb.status | domain | - |
item.grantfulltext | open | - |
item.creatorGND | Ray, Amrit | - |
item.cerifentitytype | Publications | - |
item.creatorOrcid | Ray, Amrit | - |
item.advisorGND | Renz, Wolfgang | - |
item.languageiso639-1 | de | - |
item.openairecristype | http://purl.org/coar/resource_type/c_46ec | - |
item.fulltext | With Fulltext | - |
item.openairetype | Thesis | - |
Appears in Collections: | Theses |
Files in This Item:
File | Description | Size | Format | |
---|---|---|---|---|
Amrit_Raj_2159114_Bachelor_Thesis.pdf | 3.14 MB | Adobe PDF | View/Open |
Note about this record
Export
Items in REPOSIT are protected by copyright, with all rights reserved, unless otherwise indicated.