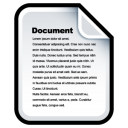
DC Field | Value | Language |
---|---|---|
dc.contributor.advisor | Neitzke, Michael | - |
dc.contributor.author | Meo, Roland | |
dc.date.accessioned | 2020-09-29T14:29:48Z | - |
dc.date.available | 2020-09-29T14:29:48Z | - |
dc.date.created | 2017 | |
dc.date.issued | 2018-01-31 | |
dc.identifier.uri | http://hdl.handle.net/20.500.12738/8222 | - |
dc.description.abstract | Diese Bachelorarbeit behandelt die Entwicklung und Optimierung eines selbständig lernenden Pacman-Agenten, da Pacman über komplexe Zustandsdaten verfügt, welche ein häu ges Problem im Maschinen Lernen darstellen. Eine typische Herangehensweise ist hierbei die Bildung von Merkmalen, eine verallgemeinerte Abstraktion der gegebenen Daten. Im Verstärkenden Lernen werden diese Merkmale genutzt umeinenWert zu berechnen der aussagt wie vorteilhaft eine Situation. Dabei werden meist Verfahren wie lineare Funktions-approximation genutzt. Alternativ wird in dieser Thesis eine andere Herangehensweise vorgeschlagen, namentlich eine Kombination aus Verstärkenden Lernen und Tiefen Lernen mit künstlichen neuronalen Netzen. Ein neuronaler Netz-agent wird implementiert und optimiert bis diese Optimierung als genügend empfunden wird. | de |
dc.description.abstract | This bachelor thesis deals with the development and the optimization of an autonomous learning Pacman-agent, since Pacman o ers high-dimensional state data, which is a common problem in machine learning. A typical approach to this problem is using features, a high-level abstraction of the given data. In reinforcement learning these features are used to calculate a value describing how bene cial a situation is by using prediction methods like linear function approximation. This thesis suggests a di erent approach by mixing reinforcement learning and deep learning via an arti cial neural network. A neural network agent is implemented then and optimized to a level deemed su cient. | en |
dc.language.iso | de | de |
dc.rights.uri | http://rightsstatements.org/vocab/InC/1.0/ | - |
dc.subject.ddc | 004 Informatik | |
dc.title | Deep Q-Learning With Features Exemplified By Pacman | de |
dc.type | Thesis | |
openaire.rights | info:eu-repo/semantics/openAccess | |
thesis.grantor.department | Department Informatik | |
thesis.grantor.place | Hamburg | |
thesis.grantor.universityOrInstitution | Hochschule für angewandte Wissenschaften Hamburg | |
tuhh.contributor.referee | Meisel, Andreas | - |
tuhh.gvk.ppn | 1011927519 | |
tuhh.identifier.urn | urn:nbn:de:gbv:18302-reposit-82240 | - |
tuhh.note.extern | publ-mit-pod | |
tuhh.note.intern | 1 | |
tuhh.oai.show | true | en_US |
tuhh.opus.id | 4168 | |
tuhh.publication.institute | Department Informatik | |
tuhh.type.opus | Bachelor Thesis | - |
dc.subject.gnd | Maschinelles Lernen | |
dc.type.casrai | Supervised Student Publication | - |
dc.type.dini | bachelorThesis | - |
dc.type.driver | bachelorThesis | - |
dc.type.status | info:eu-repo/semantics/publishedVersion | |
dc.type.thesis | bachelorThesis | |
dcterms.DCMIType | Text | - |
tuhh.dnb.status | domain | - |
item.creatorGND | Meo, Roland | - |
item.grantfulltext | open | - |
item.openairetype | Thesis | - |
item.advisorGND | Neitzke, Michael | - |
item.fulltext | With Fulltext | - |
item.languageiso639-1 | de | - |
item.cerifentitytype | Publications | - |
item.creatorOrcid | Meo, Roland | - |
item.openairecristype | http://purl.org/coar/resource_type/c_46ec | - |
Appears in Collections: | Theses |
Files in This Item:
File | Description | Size | Format | |
---|---|---|---|---|
Roland_Meo_BA_Thesis.pdf | 1.49 MB | Adobe PDF | View/Open |
Note about this record
Export
Items in REPOSIT are protected by copyright, with all rights reserved, unless otherwise indicated.