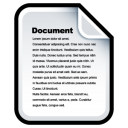
DC Field | Value | Language |
---|---|---|
dc.contributor.advisor | Neitzke, Michael | - |
dc.contributor.author | Winkler, Falco | |
dc.date.accessioned | 2020-09-29T14:44:59Z | - |
dc.date.available | 2020-09-29T14:44:59Z | - |
dc.date.created | 2018 | |
dc.date.issued | 2018-09-03 | |
dc.identifier.uri | http://hdl.handle.net/20.500.12738/8429 | - |
dc.description.abstract | Generative machine learning models make it possible to derive new data from a dataset. There are many applications in image processing, such as super-resolution and image completion. But they also find application in engineering and in other machine learning algorithms. A generative adversarial network (GAN) is trained by pitting a neural net that generates data out of a noise input, against an adversary, that tries to discriminate between fake and real data. This thesis is about the theory of GAN’s and their application on the datasets MNIST and CIFAR10 in an unsupervised and semi-supervised fashion. A state of the art and fully automated implementation is used to achieve high quality image generation. | en |
dc.description.abstract | Mit generierenden Machine Learning - Modellen ist es möglich, neue Daten aus einem Datensatz zu gewinnen. Es gibt viele Anwendungen in der Bildverarbeitung, wie zum Beispiel Auflösungserhöhung und Bildvervollständigung. Aber auch in der Medizin oder in anderen Machine Learning - Algorithmen finden sie Anwendung. Ein generative adversarial network (GAN) wird trainiert, indem ein neuronales Netz, welches Daten aus einem zufälligen Rauschen generiert, gegen einen Gegner ausgespielt wird. Dieser versucht dann, falsche von echten Daten zu unterscheiden. Die Arbeit handelt von der Theorie der GAN’s und deren Anwendung auf die Datensätze MNIST und CIFAR10 in sowohl unüberwachter als auch halb-überwachter Weise. Eine dem Stand der Technik entsprechende und vollautomatisierte Implementierung wird verwendet um hochqualitative Bilder zu generieren. | de |
dc.language.iso | en | en |
dc.rights.uri | http://rightsstatements.org/vocab/InC/1.0/ | - |
dc.subject.ddc | 004 Informatik | |
dc.title | Image Representation Learning with Generative Adversarial Networks | en |
dc.title.alternative | Image Representation Learning with Generative Adversarial Networks | en |
dc.type | Thesis | |
openaire.rights | info:eu-repo/semantics/openAccess | |
thesis.grantor.department | Department Informatik | |
thesis.grantor.place | Hamburg | |
thesis.grantor.universityOrInstitution | Hochschule für angewandte Wissenschaften Hamburg | |
tuhh.contributor.referee | Zukunft, Olaf | - |
tuhh.gvk.ppn | 1029992886 | |
tuhh.identifier.urn | urn:nbn:de:gbv:18302-reposit-84319 | - |
tuhh.note.extern | publ-mit-pod | |
tuhh.note.intern | 1 | |
tuhh.oai.show | true | en_US |
tuhh.opus.id | 4361 | |
tuhh.publication.institute | Department Informatik | |
tuhh.type.opus | Bachelor Thesis | - |
dc.subject.gnd | Maschinelles Lernen | |
dc.subject.gnd | Bildverarbeitung | |
dc.subject.gnd | Automation | |
dc.type.casrai | Supervised Student Publication | - |
dc.type.dini | bachelorThesis | - |
dc.type.driver | bachelorThesis | - |
dc.type.status | info:eu-repo/semantics/publishedVersion | |
dc.type.thesis | bachelorThesis | |
dcterms.DCMIType | Text | - |
tuhh.dnb.status | domain | - |
item.grantfulltext | open | - |
item.creatorGND | Winkler, Falco | - |
item.cerifentitytype | Publications | - |
item.creatorOrcid | Winkler, Falco | - |
item.advisorGND | Neitzke, Michael | - |
item.languageiso639-1 | en | - |
item.openairecristype | http://purl.org/coar/resource_type/c_46ec | - |
item.fulltext | With Fulltext | - |
item.openairetype | Thesis | - |
Appears in Collections: | Theses |
Files in This Item:
File | Description | Size | Format | |
---|---|---|---|---|
bachelor_thesis.pdf | 5.13 MB | Adobe PDF | View/Open |
Note about this record
Export
Items in REPOSIT are protected by copyright, with all rights reserved, unless otherwise indicated.