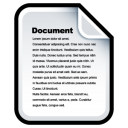
DC Element | Wert | Sprache |
---|---|---|
dc.contributor.advisor | Schiemann, Thomas | - |
dc.contributor.author | Hristova, Evelin Vladislavova | |
dc.date.accessioned | 2020-09-29T15:02:44Z | - |
dc.date.available | 2020-09-29T15:02:44Z | - |
dc.date.created | 2017 | |
dc.date.issued | 2019-04-26 | |
dc.identifier.uri | http://hdl.handle.net/20.500.12738/8696 | - |
dc.description.abstract | Automated segmentation of medical image data is an important, clinically relevant task as manual delineation of organs is time-consuming and subject to inter- and intraobserver uctuations. This thesis builds upon a framework for segmentation of multiple organs in three-dimensional images. The approach employs a supervised recognition, where a training set with dense organs annotations is used to classify voxels in unseen images based on similarity of binary features extracted from the data. A combination of two di erent types of feature vectors is used to capture relevant structural and contextual information. The binary vectors are constructed by multiple pairwise intensity comparisons. Hence, the method is invariant to monotonic gray-level changes and can be applied to di erent imaging modalities or anatomies. The fast approximate nearest neighbor search, using Vantage Point Forests, does not require any explicit prior shape model knowledge and allows computationally e cient binary data classi cation. Training the algorithm takes several minutes, while segmenting a test image is in the order of a few seconds. The method is successfully applied to 68 CT abdominal and 42 MR pelvic images. With respect to ground truth, an average Dice overlap score of 0:74 for the CT segmentation of liver, spleen and kidneys is achieved. The mean score for the MR delineation of bladder, bones, prostate and rectum is 0:65. The results demonstrate surprisingly accurate segmentation, robustness and data-e ciency. | en |
dc.language.iso | en | en |
dc.rights.uri | http://rightsstatements.org/vocab/InC/1.0/ | - |
dc.subject.ddc | 570 Biowissenschaften, Biologie | |
dc.title | 3D Medical Image Segmentation with Vantage Point Forests and Binary Context Features | en |
dc.type | Thesis | |
openaire.rights | info:eu-repo/semantics/openAccess | |
thesis.grantor.department | Department Medizintechnik | |
thesis.grantor.place | Hamburg | |
thesis.grantor.universityOrInstitution | Hochschule für angewandte Wissenschaften Hamburg | |
tuhh.contributor.referee | Nickisch, Hannes | - |
tuhh.gvk.ppn | 1663681449 | |
tuhh.identifier.urn | urn:nbn:de:gbv:18302-reposit-86984 | - |
tuhh.note.extern | publ-mit-pod | |
tuhh.note.intern | 1 | |
tuhh.oai.show | true | en_US |
tuhh.opus.id | 4718 | |
tuhh.publication.institute | Department Medizintechnik | |
tuhh.type.opus | Masterarbeit | - |
dc.subject.gnd | Bildsegmentierung | |
dc.subject.gnd | Bildverarbeitung | |
dc.subject.gnd | 3D-Technologie | |
dc.type.casrai | Supervised Student Publication | - |
dc.type.dini | masterThesis | - |
dc.type.driver | masterThesis | - |
dc.type.status | info:eu-repo/semantics/publishedVersion | |
dc.type.thesis | masterThesis | |
dcterms.DCMIType | Text | - |
tuhh.dnb.status | domain | - |
item.creatorGND | Hristova, Evelin Vladislavova | - |
item.grantfulltext | open | - |
item.openairetype | Thesis | - |
item.advisorGND | Schiemann, Thomas | - |
item.fulltext | With Fulltext | - |
item.languageiso639-1 | en | - |
item.cerifentitytype | Publications | - |
item.creatorOrcid | Hristova, Evelin Vladislavova | - |
item.openairecristype | http://purl.org/coar/resource_type/c_46ec | - |
Enthalten in den Sammlungen: | Theses |
Dateien zu dieser Ressource:
Datei | Beschreibung | Größe | Format | |
---|---|---|---|---|
thesis.pdf | 8.33 MB | Adobe PDF | Öffnen/Anzeigen |
Feedback zu diesem Datensatz
Export
Alle Ressourcen in diesem Repository sind urheberrechtlich geschützt.